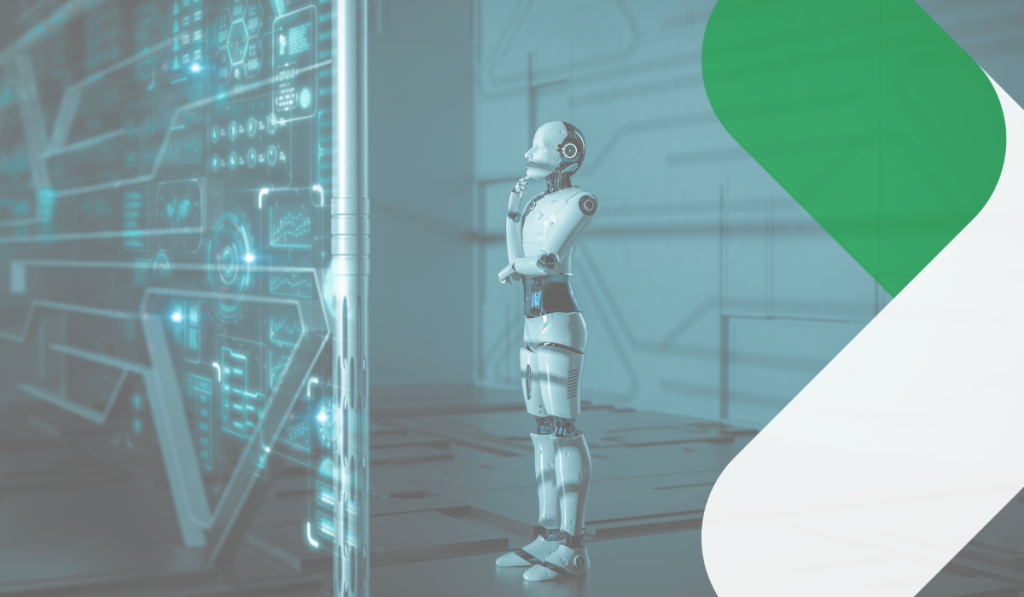
Unlocking the Future: A Comprehensive Guide to Physical AI
Exploring physical AI, delving into its main technologies, transformative applications, and implications for a smarter, more connected future.
Lately, GenAI has captured widespread attention, marked by substantial investments and a surge in technological advancements. According to a recent report by McKinsey, GenAI-powered tools could potentially halve the time it takes developers to document code functionality and double the speed at which new code can be written.
The financial figures surrounding GenAI investments are equally compelling. Billions of dollars are being funneled into GenAI startups and projects, signaling a strong belief in its transformative potential. According to E&Y, productivity driven by GenAI is poised to significantly enhance economic performance, potentially adding $650 billion to the US economy within the next decade and increasing real US GDP by approximately 2.5% by 2033. If total factor productivity grows at a rate 50% faster than the pace observed from 2017 to 2022, the surge in productivity facilitated by GenAI is expected to contribute an extra 0.25 percentage points annually to US GDP growth over the forthcoming decade.
Moreover, Gartner predicts that the rapid adoption of GenAI and the increasing expectations for sustainable business practices will intensify competition among tech suppliers, suggesting a significant shift in how software solutions are developed and delivered.
Within the fervor and innovation of GenAI, a crucial question arises: What are the real costs of building GenAI-based products? The excitement masks a landscape filled with financial, operational, and strategic challenges that need careful navigation to fully exploit GenAI’s potential.
This article delves into the multifaceted costs of GenAI projects, uncovering not just the obvious expenses but also hidden ones, alongside strategic considerations for businesses entering this promising yet demanding field.
The costs associated with GenAI-powered software development are substantial and diverse. However, when strategically managed, these investments can produce considerable efficiencies, innovations, and competitive advantages, aligning with broader market trends toward automation, intelligence, and personalized user experiences in software development.
The journey toward leveraging GenAI begins with acquiring and preparing high-quality training data. This step is critical, as a GenAI model’s efficiency is directly tied to its training data’s quality, diversity, and relevance. The costs associated with this phase can vary widely, depending on the field and complexity of the tasks the model is expected to perform.
Data labeling and cleaning, essential for reducing model bias and improving accuracy, represent a substantial portion of these costs. Depending on the nature of your data and the complexity of your project, a solid dataset will cost anywhere from $10,500 to $85,000. Moreover, as data privacy and ethical considerations gain importance, the costs of ensuring compliance with regulations and ethical guidelines are also becoming significant factors for companies to consider.
Developing a GenAI model involves a wide array of costs, the biggest of which are computational resources. Training complex GenAI models often requires significant cloud computing power, resulting in hefty expenses, especially for models requiring multiple iterations to refine and improve. Therefore, the cost of a generative AI solution depends on whether you’re using your own hardware or cloud services, with the latter’s price varying based on the cloud provider and the scale of your operations.
If you opt for a simpler model and deploy it on-premises, you’re expected to spend $10,000–30,000 in GPU costs to fine-tune the generative AI solution. With cloud computing, the expenses could range between $1 and $10 per hour, depending on instance type. GPT-3-like open-source models require a more advanced GPU setup, upwards of $50,000–$100,000. For high-end GPU models, the associated cloud computing expenses can range from $10 to $24 per hour.
Beyond computing costs, the expertise required to develop, train, and manage GenAI models is another critical expense. The demand for professionals skilled in AI and machine learning far exceeds supply, leading to premium salaries for this talent. Artificial intelligence talent doesn’t come cheap. A US-based in-house AI engineer will cost your company $70,000–$200,000 annually, let alone the hiring, payroll, social security, and other administrative expenses. You can reduce GenAI costs by partnering with an offshore software engineering company with AI development expertise. Depending on the location, enterprise-grade offshore partners typically charge $40-$90 per hour for a software engineering talent with AI skills. However, depending on project complexity, the hourly rates for a senior data scientist or ML engineer with unique expertise can reach and exceed $200 in Europe and Latam.
Additionally, software licenses for development tools and platforms add to the financial outlay. As GenAI technologies evolve, staying at the cutting edge may need ongoing investments in training and tool upgrades, ensuring that development teams have access to the latest advancements in the field.
Integrating a GenAI model into existing software systems introduces a new set of challenges and costs. Developing robust data pipelines to feed real-time data into the model, creating APIs for model access, and ensuring the GenAI components work seamlessly with legacy systems are all crucial steps that require careful planning and investment. PwC highlights GenAI’s transformative potential in enhancing capacity, productivity, and augmentation, suggesting that these investments can lead to substantial long-term benefits for organizations.
Integrating the AI model into your existing systems and deploying it (especially in a production environment) requires additional software development efforts, which means labor costs entail substantial outlays for employing skilled developers. Given the advanced nature of GenAI integration, the demand for expertise in this niche significantly increases the project’s labor costs. This need for skilled labor spans across the development, integration, and iteration phases, underscoring the importance of strategic planning and investment in human resources to ensure the successful deployment and operation of GenAI technologies within existing software ecosystems.
Embarking on GenAI-powered software development projects opens the door to innovation and efficiency. Yet, it also presents a series of financial considerations that extend beyond the surface. The temptation to automate and enhance development processes with GenAI is compelling, but it’s accompanied by hidden costs that organizations must navigate to fully leverage this technology.
The necessity for human expertise in the GenAI landscape cannot be understated. While GenAI promises automation and efficiency, skilled professionals are vital in guiding, managing, and interpreting GenAI systems. The demand for AI, data science, and machine learning experts has skyrocketed, leading to a competitive job market with substantial salaries. According to the World Economic Forum, AI and machine learning roles are among the most in-demand in the technology sector, reflecting the critical need for human oversight in these advanced systems.
The size of the team directly influences the development costs of GenAI projects due to the necessity of hiring skilled professionals. With the demand for AI expertise rising, salaries for these roles are significant, ranging from $80,000 to $150,000 annually. A small project may only require a few specialists, but larger endeavors need a diverse team, including data scientists, AI engineers, developers, UX/UI designers, compliance experts and PMs. Thus, the broader the team’s scope, the higher the overall project cost, aligning with the need for comprehensive skills to manage, develop, and deploy GenAI solutions effectively.
Beyond the cost implications of hiring and retaining top talent, there’s also the investment in continuous education and training. GenAI is evolving rapidly, needing ongoing learning and professional development to keep pace with new methodologies, tools, and best practices. These training programs represent a significant investment but are essential for maintaining a skilled workforce capable of leveraging GenAI to its fullest potential.
The challenge of bias in GenAI models is a critical concern with financial, ethical, and legal implications. Making sure that GenAI systems operate fairly and without prejudice requires extensive testing and refinement, often involving collecting and annotating additional data to balance and diversify training datasets. Mitigating bias can be substantial, as it involves technical adjustments and consultations with experts in ethics, legal compliance, and domain-specific knowledge to examine and address potential biases.
Explainability, or the ability of GenAI systems to justify their decisions in understandable terms, is equally important. Regulations such as the UK’s General Data Protection Regulation (UK GDPR) have introduced requirements for transparency in automated decision-making, compelling organizations to invest in explainability frameworks. Developing these systems to meet regulatory standards and build user trust involves additional complexity and expense, often requiring interdisciplinary teams of legal experts, data scientists, and user experience designers.
The iterative nature of GenAI project development incorporates a trial-and-error process that, while essential for innovation, carries underlying costs. Experimentation is at the heart of GenAI’s promise, allowing developers to explore novel applications and refine models for optimal performance. However, this quest comes with the risk of failures and missteps, which, although valuable learning opportunities, represent time and resources that could significantly impact project budgets.
Failed experiments and the need for adjustments can escalate project costs, particularly regarding computational resources and human capital. There have been some talks about adopting an agile approach to GenAI projects while mitigating some risks of trial and error. However, careful financial planning and management are still required to balance innovation with cost-effectiveness.
As organizations navigate the complex landscape of GenAI-powered software development, a key question arises: Do GenAI’s benefits outweigh its costs? This question is especially relevant in an era of peak technological innovation and market competition. Balancing GenAI’s costs against its potential benefits is critical for companies looking to invest in this technology.
The initial financial investment for GenAI projects includes costs associated with data acquisition, computational resources, human expertise, and integration into existing systems. Despite these expenses, GenAI promises significant ROI through increased efficiency, productivity gains, and the creation of innovative products and services. A McKinsey study on the impact of AI technologies suggests that GenAI can drive substantial improvements in efficiency and cost savings across various industries, with productivity gains potentially boosting global GDP by up to 1.2% annually.
Beyond financial metrics, GenAI’s impact on operational efficiency and competitiveness is profound. Companies leveraging GenAI can automate routine tasks, accelerate development cycles, and enhance decision-making processes. This operational agility allows businesses to respond more quickly to market changes and customer needs, thereby gaining a competitive edge. However, integrating GenAI systems into existing operational frameworks can be complex and time-consuming, requiring careful planning and execution.
Strategically, GenAI offers the potential to redefine market paradigms and create new business models. For instance, GenAI’s ability to generate personalized content and recommendations can open new avenues for customer engagement and revenue generation. However, adopting GenAI also needs a strategic shift towards a more agile, innovation-driven culture capable of managing the pace of change and ethical considerations associated with AI technologies.
The global AI market, including GenAI, is experiencing rapid growth. Forecasts suggest it could reach $190.61 billion by 2025. This growth indicates the increasing adoption of AI technologies across sectors, driven by the need for automation and smart systems in the face of rising operational complexities and data volumes.
Whether GenAI suits your organization requires a comprehensive examination of costs, benefits, and strategic goals. Organizations must carefully consider their readiness to adopt GenAI, including the financial capacity, operational agility, and strategic vision to leverage this technology effectively. As the landscape of GenAI continues to evolve, staying informed and adaptable will be vital to harnessing its potential for driving business success in the digital era.
Tracking the Return on Investment (ROI) for GenAI projects is an essential aspect that organizations must rigorously manage to ensure their initiatives’ financial viability and strategic success. Quantifying the actual benefits becomes vital given the substantial investments required for GenAI development, from data acquisition and model training to integration and maintenance. This assessment enables companies to make informed decisions about future investments in GenAI technologies and adjust their strategies accordingly.
The ROI of GenAI projects can be measured through various metrics, reflecting the multiple benefits these technologies offer. Key performance indicators (KPIs) include:
For instance, operational efficiency can be measured by reducing time-to-market for new software products or enhancements. At the same time, automation-driven cost savings may be quantified by the decrease in manual labor hours required for specific tasks.
Market trends indicate a growing recognition of GenAI’s strategic value across industries. The AI market’s rapid expansion, forecasted to reach billions in the coming years, highlights the potential for significant ROI for early adopters who successfully integrate GenAI into their operations. Companies that leverage GenAI to innovate in product development, customer service, and operational processes not only stand to gain immediate financial returns but also position themselves competitively for future growth.
However, accurately measuring ROI involves challenges, particularly in attributing specific financial gains to GenAI projects amongst the numerous factors affecting business performance. Organizations must adopt robust methodologies for tracking and analyzing the impact of GenAI initiatives, incorporating quantitative and qualitative data to capture the full spectrum of benefits. This might involve advanced analytics to link GenAI use with performance improvements, customer surveys to assess satisfaction levels, and case studies to document operational efficiencies gained through automation.
In addition to direct financial returns, companies should also consider GenAI’s broader impacts on their strategic objectives, such as enhancing innovation capabilities, attracting top talent, and strengthening their brand reputation as technology leaders. While more challenging to quantify, these strategic advantages are crucial to long-term business success and must be factored into the overall ROI analysis.
As companies worldwide continue to explore and expand their GenAI capabilities, the narrative is no longer about whether to invest in GenAI but how to do so wisely, ethically, and effectively. The journey towards GenAI-powered innovation involves significant costs and complex ethical dilemmas. Yet, with careful planning, comprehensive ROI tracking, and a steadfast commitment to leveraging technology for the greater good, the potential rewards for businesses, their customers, and society are unparalleled.
Organizations must remain vigilant, adaptable, and ethically grounded as we move ahead into a future increasingly shaped by GenAI. While the costs associated with GenAI development are substantial, they represent an investment in a more intelligent, efficient, and potentially more equitable world. By embracing GenAI with a balanced perspective on its costs, benefits, and broader implications, companies can lead toward a future where technology amplifies human potential and drives sustainable growth.
Exploring physical AI, delving into its main technologies, transformative applications, and implications for a smarter, more connected future.
Examining the challenges of data black holes and providing a practical tips for organizations seeking to harness the full potential of their data ecosystems.
This article addresses how AI technologies are transforming predictive maintenance in manufacturing.