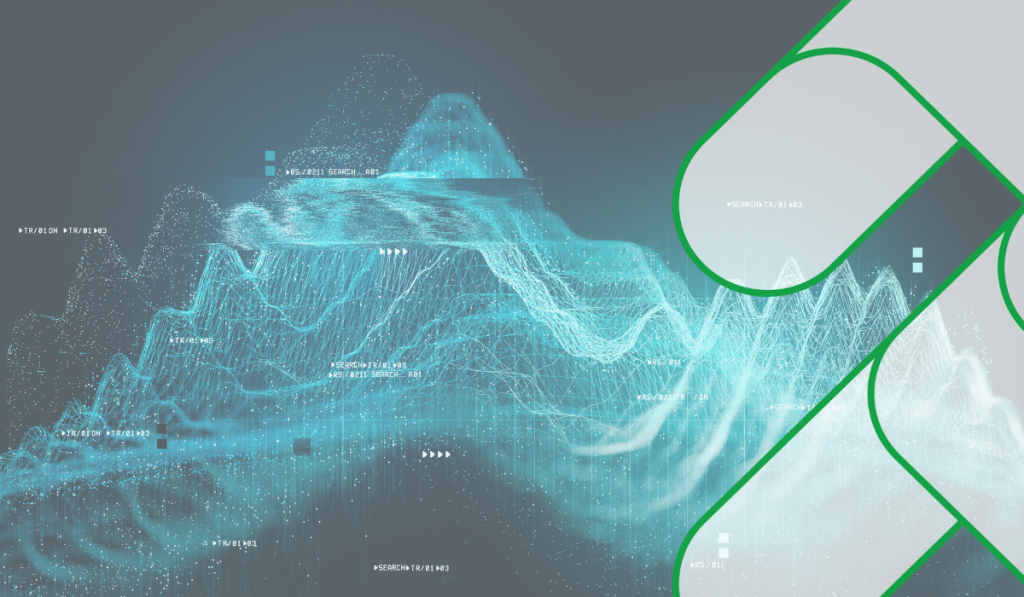
How AI Turns Data Black Holes Into Actionable Insights In Supply Chain
Examining the challenges of data black holes and providing a practical tips for organizations seeking to harness the full potential of their data ecosystems.
The global AI robotics market is constantly growing, with forecasts predicting 1.3 billion AI robots in operation by 2035 and an astonishing 4 billion by 2050. The main drivers of this sector are advancements in AI technology, economic issues such as labor shortages, and a focus on betterment through automation, including household and industrial jobs. These advancements signify a pivotal moment in technological evolution, where intelligent machines extend their reach beyond the digital realm to reshape real-world applications.
As the world increasingly adopts technologies like autonomous vehicles, AI-powered surgical tools, and adaptive manufacturing systems, physical AI stands at the forefront of this revolution. Its influence spans industries, addressing complex challenges and unlocking unprecedented efficiencies.
This article explores physical AI, delving into its main technologies, transformative applications, and implications for a smarter, more connected future.
Physical AI integrates artificial intelligence with physical hardware and systems, enabling machines to interact autonomously with the physical world. It represents a profound leap from traditional systems by incorporating advanced decision-making capabilities directly into devices that can manipulate and adapt to their surroundings. Unlike conventional robotics, which often rely on pre-programmed instructions, physical AI leverages real-time data and advanced learning techniques such as neural networks, reinforcement learning, and adaptive algorithms to analyze inputs and respond dynamically. This enables systems to make predictive adjustments, optimize operations over time, and effectively adapt to ever-changing environmental variables. Ensuring greater flexibility and efficiency in its operations.
Sensors and actuators form the foundation of physical AI by bridging the gap between the digital and physical realms. For instance, LIDAR, a key sensor technology, enables robots to detect and navigate objects in real time, processing 3D signals tens of times faster than humans. This level of precision ensures that robots can safely and effectively interact with complex stimuli in real time, paving the way for innovations like autonomous vehicles and robotic surgery.
Control systems are integral to coordinating the complex functions of physical AI devices. These systems manage the flow of information between sensors, processors, and actuators, ensuring synchronized operations. Using advanced algorithms, control systems enable machines to perform precise and adaptive actions, whether a robotic arm assembling intricate components or a drone navigating unpredictable terrain. This orchestration enhances both the reliability and efficiency of physical AI technologies.
AI algorithms are the brains of physical AI, allowing machines to analyze data, make decisions, and learn from their experiences. These algorithms utilize machine learning, neural networks, and reinforcement learning techniques to interpret sensor data and optimize performance. For instance, in autonomous systems, AI algorithms process vast amounts of environmental data to predict obstacles and adjust actions accordingly. Their continuous evolution ensures that physical AI systems remain adaptive and capable of tackling increasingly complex tasks.
Sensors are the eyes and ears of physical AI, enabling machines to perceive and interpret the world around them. Modern sensors, such as LIDAR, ultrasonic devices, infrared systems, and high-resolution cameras, gather critical data about environmental conditions, distance, motion, and object characteristics. These technologies ensure that physical AI systems operate with precision and situational awareness, which is essential for safety and efficiency in real-world applications.
At the heart of physical AI lies the ability to process data and make smart decisions made possible by machine learning and advanced AI algorithms. These algorithms analyze sensor inputs and predict optimal actions, enabling machines to adapt to dynamic environments. Neural networks, for instance, empower systems to identify patterns and recognize objects, while reinforcement learning enhances decision-making in uncertain scenarios. These technologies allow physical AI devices to evolve and improve, addressing complex challenges with minimal human intervention.
Robotics is the physical embodiment of AI, combining mechanical engineering with computational intelligence. Technological advancements have created dexterous robotic arms, agile drones, and humanoid robots capable of mimicking human-like movements. These developments, fueled by improved materials and precise actuators, enable robots to perform intricate tasks such as assembling microelectronics or conducting minimally invasive surgeries. Robotics amplifies the capabilities of physical AI, bridging the gap between digital intelligence and tangible action.
Edge computing has become a foundation of physical AI, enabling real-time data processing directly on devices rather than relying on distant cloud servers. For example, autonomous vehicles use edge computing to process inputs such as LIDAR data and camera feeds, allowing split-second decisions that enhance safety and operational efficiency. Additionally, advancements in neural processing units (NPUs) have accelerated AI computations, ensuring that devices can operate in environments with limited connectivity.
Physical AI is driving innovation in manufacturing by introducing adaptive robots capable of transforming assembly lines into highly efficient and responsive systems. These robots leverage AI to adjust their actions in real time based on changes in production requirements, significantly reducing downtime and errors. Additionally, vision systems with AI algorithms enhance quality control by accurately detecting defects, ensuring consistent product standards, and reducing waste.
Physical AI is revolutionizing patient care and medical procedures in healthcare. Surgical robots with advanced AI algorithms perform complex operations with a precision that surpasses human capabilities, reducing complications and recovery times. Moreover, wearable health monitors integrated with physical AI enable continuous tracking of vital signs, allowing for early detection of medical issues and personalized treatment plans. This integration empowers healthcare professionals to make more informed decisions, ultimately improving patient outcomes.
Transportation systems are being transformed by integrating physical AI, particularly through the development of autonomous vehicles. For example, Waymo’s autonomous vehicles have shown that over 7.1 million miles driven, there were an estimated 17 fewer injuries and 20 fewer police-reported crashes compared to the expected outcomes if human drivers, using the benchmark crash rate, had driven the same distance in the same areas. These systems use AI to navigate complex environments, making real-time decisions to ensure safety and efficiency. Additionally, physical AI enhances traffic management systems by analyzing and optimizing traffic flow, reducing congestion and emissions. This evolution in transportation is paving the way for safer and more sustainable mobility solutions.
Physical AI is addressing the challenges of modern agriculture by enabling precision farming techniques. AI-powered drones monitor crop health, soil conditions, and irrigation needs, providing farmers with actionable insights to optimize yields. Smart machinery with sensors and AI automates planting, harvesting, and maintenance tasks, reducing resource waste and labor costs. These advancements are helping to meet the growing demand for food while promoting sustainable agricultural practices.
Physical AI enhances convenience and efficiency through smart home devices and personal assistants in everyday life. For example, domestic cleaning robots, a mature market rapidly expanding globally, are anticipated to reach around $48.45 billion by 2034. These devices have advanced features such as vision-based navigation, enabling them to recognize and avoid obstacles while maintaining cleaning efficiency. These technologies are redefining how we interact and benefit from our living environments.
Physical AI faces significant technical challenges, with real-time data processing standing out as a major limitation. Many applications require the instantaneous interpretation and analysis of vast amounts of data, pushing the limits of existing processing technologies.
Another critical issue is ensuring the reliability of AI-driven decisions in dynamic and unpredictable environments. Machines must be capable of making accurate, split-second decisions, especially in high-stakes applications such as healthcare and transportation. Additionally, integrating diverse hardware and software systems often leads to compatibility and operational challenges, hindering seamless performance.
The ethical implications of physical AI are profound and multifaceted. Privacy concerns stem from the extensive data collection needed to train and operate AI systems, raising questions about how this data is stored, shared, and used. The interconnected nature of physical AI systems also introduces significant security risks, as vulnerabilities can be exploited to cause widespread harm. Furthermore, debates around the autonomy of AI in decision-making highlight ethical dilemmas, particularly when AI systems must make life-altering choices. Addressing these issues requires robust frameworks to ensure accountability, fairness, and transparency.
Despite its potential, the widespread adoption of physical AI is constrained by high initial costs. Developing and deploying advanced AI systems, including sensors, processors, and actuators, demands significant financial investment. These costs pose barriers for smaller organizations and industries with limited budgets. Scalability also presents a challenge, as adapting physical AI systems to diverse operational environments often requires extensive customization. Overcoming these financial and technical barriers is essential to making physical AI accessible and practical on a larger scale.
Establishing effective and intuitive human-AI interaction is crucial for the acceptance and success of physical AI systems. Many users remain skeptical of AI, particularly in applications where machines replace human roles or make independent decisions. Building trust in AI systems requires clear communication about their capabilities, limitations, and benefits. Ensuring that AI systems are user-friendly and aligned with human values will promote integration into daily life and foster societal acceptance.
ERIS, a state-of-the-art robotic system developed by our scale-up Adapta Robotics, demonstrates the potential of physical AI by combining advanced AI algorithms with dexterous hardware.
This robot is designed for retail environments, excelling in automated shelf monitoring and price tag issues.
Equipped with a custom-developed neural network, ERIS efficiently processes high-resolution images and detects price tags displayed on shelves. Each identified label is passed through artificial intelligence algorithms, extracting product prices and barcode locations. This allows ERIS to determine price tag errors on paperback and electronic labels and report when prices must be rectified.
ERIS demonstrates how physical AI can revolutionize the retail industry by streamlining operational efficiency and reducing manual errors. By showcasing these capabilities, ERIS serves as a benchmark for future applications of physical AI in complex and fast-paced environments.
The future of physical AI is closely tied to advancements in complementary technologies such as 5G, quantum computing, and advanced materials. Implementing 5G networks will significantly enhance the speed and reliability of data transfer, allowing physical AI systems to function more effectively in real-time environments. Similarly, breakthroughs in quantum computing could unlock unprecedented computational power, enabling physical AI to solve complex problems with greater accuracy and efficiency. In addition, developing lightweight and durable materials will pave the way for more versatile and cost-effective AI-powered machines.
Integrating physical AI with other emerging technologies will open new possibilities across industries. For example, combining physical AI with blockchain technology can enhance the security and transparency of data exchange in autonomous systems. Combining physical AI and bioengineering in healthcare could lead to the development of advanced prosthetics and personalized medical devices. Furthermore, integrating AI with renewable energy technologies could optimize energy consumption in smart cities, promoting sustainability on a global scale.
As physical AI continues to evolve, its impact on industries will become increasingly profound. Manufacturing will see the emergence of “smart factories” where interconnected AI-driven systems operate with minimal human intervention. Transportation networks will transform into intelligent ecosystems integrating autonomous vehicles, drones, and public transit systems. AI-powered machines and systems will redefine food production in agriculture by increasing efficiency and reducing environmental impact. These transformations will reshape industries, creating new business models and opportunities.
The advancement of physical AI will necessitate the establishment of robust ethical and regulatory frameworks to address privacy, security, and accountability concerns. Governments and organizations must collaborate to create guidelines ensuring the responsible development and deployment of physical AI systems. These frameworks should emphasize fairness, transparency, and inclusivity, fostering public trust and encouraging widespread adoption. Additionally, ongoing dialogue with stakeholders, including industry leaders, policymakers, and the public, will be essential in shaping regulations that adapt to evolving technological landscapes.
Physical AI represents a groundbreaking evolution in technological innovation, poised to redefine how industries operate, and individuals engage with technology. Its capacity to merge intelligent algorithms with physical systems opens unprecedented possibilities, fostering smarter solutions across healthcare, manufacturing, transportation, and beyond. However, achieving its full potential requires addressing the inherent challenges, including technical limitations, ethical concerns, and cost barriers. As we look ahead, the synergy between physical AI and emerging technologies like 5G, quantum computing, and renewable energy will drive the next wave of innovation.
For companies eager to embrace the future, partnering with technology accelerators like rinf.tech offers a unique opportunity to harness the transformative power of physical AI. Organizations can unlock unprecedented growth, efficiency, and impact with expert guidance and tailored solutions. The era of physical AI has begun, and those who act decisively will lead the way into a smarter, more connected world.
Let’s talk.
Examining the challenges of data black holes and providing a practical tips for organizations seeking to harness the full potential of their data ecosystems.
This article addresses how AI technologies are transforming predictive maintenance in manufacturing.
Exploring how AI and Blockchain technologies complement each other, demonstrating their unique advantages and showcasing their profound implications for multiple industries.