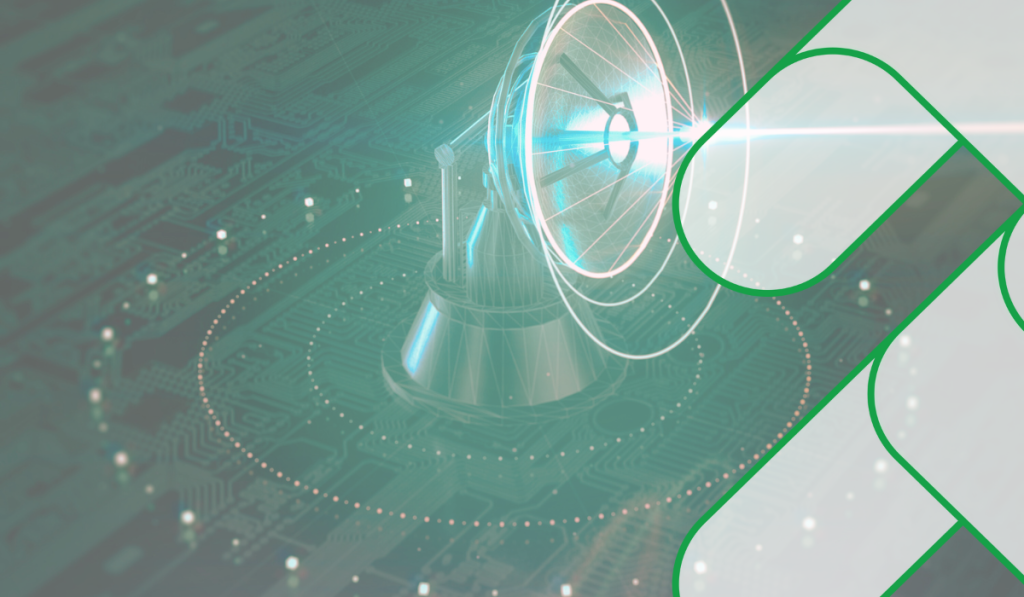
AI-Powered LiDAR: A New Era of Perception
How AI and ML enhance LiDAR technology by overcoming data processing challenges, enabling advanced applications in autonomous systems, robotics, and 3D mapping, and more.
ML use cases across industries can vary greatly. Identifying a sector that wouldn’t benefit from machine learning projects would be difficult. However, some sectors would certainly benefit more than others. They include retail, transportation, delivery and supply chain, semiconductor, and hardware.
This article explores interesting machine learning projects that companies in various sectors can launch to gain a significant competitive advantage in a highly saturated business landscape. The slightest advantage via efficient and streamlined operations can nudge a company ahead of its competitors, and certain ML ideas can provide a massive headstart.
The best machine learning ideas can benefit organizations in the abovementioned industries, including higher cost-savings, increased revenue, greater operational efficiency, better safety, security, and compliance, optimized workforce and resource utilization, and improved human and machine behavior pattern recognition accuracy. Let’s explore some ML project ideas for different industries.
The retail industry has tremendous potential to benefit from advanced ML. ML has already made significant inroads in retail. The best ML use in retail includes cutting-edge recommendation engines, fraud detection, virtual assistants and chatbots, highly personalized targeted ads, and video surveillance.
ML in video surveillance for retail environments has exciting possibilities, and that’s best exhibited in rinf.tech’s Vid.Supervisor machine learning model. Vid.Supervisor runs over videos in retail stores to identify and tag human behaviors without breaching their confidentiality—the core features of Vid.Supervisor include people detection, facial recognition, and automatic video creation.
rinf.tech’s AR (augmented reality) shopping app is another example of ML potential in retail. The innovation was in the form of a mobile application that helped customers identify products in a store and automatically add them to a digital shopping cart or bookmark them to be shared later. This project is a reminder that ultramodern technologies like ML can augment and co-exist with traditional in-store shopping experiences that many global consumers still prefer.
Tremendous ML activity and advancements are occurring in transportation sectors around the world. Businesses are facing significant transportation-related challenges that could result in financial setbacks if left unaddressed. These challenges include centralized management of large fleets, avoiding empty miles, and ever-increasing costs of labor, fuel, equipment, and vehicles.
ML use in transportation can provide several benefits via route optimization, predictive fleet maintenance, computer vision-based smart parking solutions, self-driving vehicles, pedestrian detection, and traffic management.
rinf.tech developed Cloud CCTV Services for trains. Developed for AdComms, a key supplier across the rail industry in the UK, this project was a response to AdComms’ request for an enterprise-grade Cloud solution to facilitate remote access to CCTV video footage on trains in the UK.
We at rinf.tech responded with an ML-powered four-pronged solution that comprised on-train Windows services, cloud services, a web application, and an SPSO-driver application. This highly lauded and awarded CCTV solution is currently running on UK trains, a monument to the potential of ML use in transportation.
The delivery and supply chain industry, one of the major engines of the world’s economic growth, faces a series of challenges, including labor shortages, material scarcity, equipment availability, forecasting complexities, port congestion, energy scarcity, and repercussions of various global bottlenecks. The practical application of ML can solve many of these challenges and shape a new delivery and supply chain management era.
Some examples of ML solutions that can transform delivery and supply chain activities across the globe include robust automated warehouse management, advanced IoT (internet-of-things) machines and vehicles, robotic process automation, computer vision-powered smart surveillance systems, anomaly detection, and highly accurate demand forecasting.
Digital twin modeling is an ML-powered technology that allows companies to recreate a digital version of a physical object or environment with real-time behavior synchronization via sensors. Creating digital twin supply chains can help companies experiment with different workflows and pipelines and try to optimize certain aspects of a supply chain process. Digital twins in the supply chain sector can help organizations improve visibility, assess risks associated with a variety of potential decisions, and simulate disasters to test out remediation playbooks.
McKinsey refers to the current decade as the “semiconductor decade.” The semiconductor industry is a global machine that’s set to become worth a trillion dollars by 2030. ML use in the semiconductor space can further drive that growth.
Semiconductors become smaller and smaller with every passing year. At the same time, companies in the semiconductor industry have mountains of data documenting the entire semiconductor technology lineage. This data can be leveraged with ML to discover new semiconductor materials and innovate ways to transform semiconductor manufacturing.
Other ideas for ML application in the semiconductor industry include smart neuromorphic chips, automated hardware design, fault detection via nano-scale image generation, streamlined manufacturing pipelines, and automated testing.
Companies are in a neck-and-neck race in this highly lucrative sector. Machine learning capabilities can provide some of the most promising companies with the deserved boost they need to get ahead of the rest and further revolutionize the industry.
Machine Learning is taking over the world. Some industries, in particular, are set to benefit greatly from the constantly-advancing powers of ML. The retail, transportation, supply chain, and semiconductor industries stand out as high-potential beneficiaries. These industries have already undergone profound change due to ML. However, these changes will likely be small stepping stones that lead to an ML-powered smart future.
ML is also rapidly transforming leading industries like healthcare, education, and energy. ML is evolving so quickly that it’s difficult to predict what effect this soon-to-be trillion-dollar industry could have on our world. One thing is certain: most modern companies, however big or small, can benefit from ML. As with most technologies, the key to success is strategic and responsible application and collaboration with experts.
Experts like rinf.tech can help modern businesses deal with vast volumes of data efficiently and effectively. That data is of no use if it can’t be mined for business insights at sub-second speeds. Our experts can help a diverse range of enterprises unlock the potential of ML and improve the quality of business insights, and optimize performance, costs, and operations.
Contact us now to learn how to do a machine-learning project to solve your most pressing business challenges.
How AI and ML enhance LiDAR technology by overcoming data processing challenges, enabling advanced applications in autonomous systems, robotics, and 3D mapping, and more.
Delving into Physical AI’s main technologies, transformative applications, and implications for a smarter, more connected future.
Examining the challenges of data black holes and providing a practical tips for organizations seeking to harness the full potential of their data ecosystems.