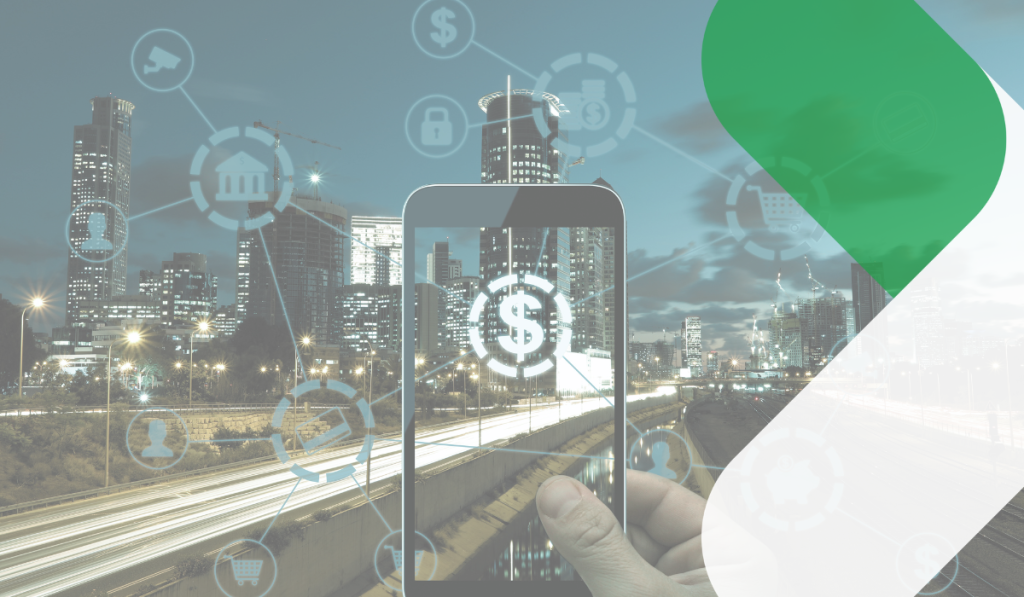
Unleashing the Power of Data: The API Economy and Open Banking
Exploring the immense potential of data-driven innovation within the financial services industry.
As AI continues to integrate deeper into financial services, there is a growing trend towards developing and implementing localized AI models and data pipelines. These localized models cater to specific applications and industries’ unique needs, such as fintech, healthcare, and insurance. This approach ensures greater flexibility, privacy, and compliance, enabling organizations to maximize the value of their AI investments. Custom localized AI models are particularly important in addressing the challenges of data security and regulatory compliance, which are critical in the financial sector. The global Generative AI market in financial services was valued at $1.19 billion in 2023 and is projected to reach $13.33 billion by 2033. This explosive growth highlights the immense potential of customized AI solutions tailored to specific challenges and opportunities in financial services.
This article explores the benefits and challenges of implementing local AI models in financial services and highlights their transformative impact on the industry. By understanding these dynamics and leveraging AI to drive innovation and growth, financial institutions can better navigate the evolving landscape.
Custom localized AI models are specialized artificial intelligence systems designed to cater to the unique needs of specific geographic regions, industries, or regulatory environments. Unlike generic AI models that are often trained on broad, global datasets, custom localized AI models are developed with a focus on the local context in which they will be used. This localization ensures that the AI systems are more accurate, relevant, and compliant with local norms, regulations, and user behaviors.
These models are trained on data that is specific to a particular region, including local languages, dialects, cultural references, and consumer behaviors. This makes them more effective in delivering relevant and understandable results within the local context.
Custom localized AI models are designed with local laws and regulations in mind, ensuring that they adhere to regional compliance requirements such as data protection laws (e.g., GDPR in Europe) and industry-specific standards.
By processing data locally and avoiding unnecessary data transfers across borders, these models help in maintaining higher levels of data privacy and security, which is especially critical in regions with stringent data protection laws.
These models are crafted to be sensitive to the region’s cultural nuances and societal norms, providing more appropriate and contextually aware responses and actions.
Beyond geographic localization, these models can also be customized for specific industries, incorporating sector-specific data and addressing particular challenges faced within that industry.
By leveraging custom localized AI models, organizations can optimize their AI strategies to be more in tune with the specific needs of their target markets, thereby driving greater value and ensuring compliance with local standards.
Relying on generic, cloud-based AI models has traditionally been the norm, but this is changing to a more localized and powerful solution: customized local AI models. These on-premises models offer a multitude of advantages and are revolutionizing financial services.
Financial data is among the most sensitive information globally, with a 2023 IBM report revealing that the financial services industry experienced the highest cost of data breaches at a staggering $5.9 million per incident. By keeping this sensitive data on-premise, local models provide a reassuring layer of protection, significantly reducing the risk of breaches and unauthorized access. Moreover, local processing ensures compliance with ever-evolving data privacy regulations like GDPR and CCPA, which impose strict controls on financial organizations’ handling of customer information. This focus on security and privacy is a crucial advantage of customized local AI models, instilling confidence in financial institutions and their customers.
Generic AI models are trained on broad datasets that may not accurately reflect the specific nuances of an individual organization’s financial data. On the other hand, local models are built and trained using an organization’s historical data and customer information. This hyper-focused approach significantly improves accuracy in fraud detection, credit risk assessment, and algorithmic trading tasks. Furthermore, local processing eliminates reliance on cloud infrastructure, often resulting in faster response times and real-time insights that can give institutions a crucial edge in the competitive financial landscape.
Cloud-based AI solutions often come with substantial subscription fees and ongoing maintenance costs. However, local models can greatly reduce these operational costs, freeing up resources for other strategic initiatives. A recent Accenture report estimates that AI can generate a potential global economic impact of $15.7 trillion by 2030, with a significant portion of these savings coming from reduced operational overhead. For specific, high-volume tasks within financial services, local models offer a cost-effective and efficient alternative to cloud-based solutions. This potential for significant cost savings and economic impact should serve as a strong motivator for financial institutions to consider adopting customized local AI models.
With local AI models, financial institutions have full control over the development and deployment processes. This means that models can be tailored to meet the specific needs of the organization, including adapting to regional market conditions, regulatory requirements, and unique business strategies. This level of customization is often difficult to achieve with off-the-shelf cloud solutions. Additionally, having control over the model’s lifecycle allows for more frequent updates and fine-tuning, ensuring the AI remains aligned with the organization’s evolving goals and the changing financial landscape.
Data sovereignty is a critical concern for financial institutions operating across multiple jurisdictions. Local AI models help ensure that data remains within the geographical boundaries required by local laws, reducing the complexity and risks associated with cross-border data transfers. This is particularly important in regions with stringent data localization laws, where failure to comply can result in heavy penalties. By leveraging localized AI, financial institutions can maintain compliance while still harnessing the full power of AI technologies.
Localized AI models empower financial institutions to innovate more rapidly by allowing them to experiment with new algorithms and approaches tailored specifically to their market and customer base. This ability to rapidly iterate and refine AI models enables organizations to stay ahead of competitors who may be relying on more generalized, slower-to-adapt cloud-based solutions. The agility offered by localized AI models can be a key differentiator in a highly competitive industry where speed and precision are critical.
Implementing custom localized AI models offers significant advantages, but it’s not without challenges. Here are some key limitations and potential solutions.
Building and maintaining effective local AI models requires a deep understanding of AI/ML technologies. A recent Glassdoor study indicates that the average salary for AI engineers in the financial services industry in 2024 is $156,831/year in the US. This high demand and a limited talent pool can make it difficult for some institutions to build their in-house AI teams.
Partnering with AI experts is a strategic approach. Many established tech companies and consultancies offer comprehensive AI development and deployment services tailored to the financial sector. This allows institutions to leverage the expertise of seasoned professionals without the burden of building an entirely new internal team.
Training powerful AI models often requires vast amounts of high-quality data. However, financial institutions may need more historical data or data siloed across different systems, restricting the model training process. A study found that 88% of all data integration projects fail entirely or significantly overrun their budgets because of poor data quality. This highlights the critical importance of robust data management and integration strategies to ensure the availability of comprehensive and clean datasets. Addressing these challenges is essential for financial institutions to fully leverage AI’s potential and achieve successful outcomes in their AI initiatives.
Techniques like data augmentation, which involves generating synthetic data points from existing data, can help address this challenge. Additionally, transfer learning, a method where a pre-trained model is adapted to a new task, can leverage knowledge from existing datasets to improve the performance of local models with smaller datasets.
While local models eliminate reliance on external cloud resources, some complex AI applications may still require significant processing power. Training large neural networks, for instance, can require powerful hardware like GPUs or TPUs. The global AI infrastructure market is expected to reach $96.6 billion by 2027. This growth points out the increasing demand for advanced computing resources, highlighting the critical role of robust and scalable infrastructure in supporting the development and deployment of AI technologies. As AI continues to evolve, balancing the benefits of local models with the need for powerful processing capabilities will remain a key consideration for organizations.
Investing in specialized hardware like Tensor Processing Units (TPUs) can provide the necessary processing power for computationally intensive tasks. Additionally, cloud bursting, where workloads are temporarily offloaded to the cloud during peak processing periods, can be a viable option for managing surges in computational demand.
Customized local AI models are poised to revolutionize various aspects of financial services, creating a more secure, efficient, and intelligent financial landscape.
Here’s a closer look at how these models are transforming key areas.
Fraudulent transactions cost financial institutions billions of dollars annually. According to an ACI study, financial crime and fraud are projected to cost banks and financial institutions around the world $40.62 billion by 2027. Local AI models trained on historical fraud data can accurately identify suspicious patterns in real time. This allows immediate intervention and prevents fraudulent transactions, significantly reducing financial losses. For instance, JPMC utilizes AI to analyze millions of transactions daily, identifying and blocking fraudulent activity with a 99% success rate.
Traditional credit scoring methods often rely on generic factors that may not accurately reflect an individual’s financial situation. Local AI models can analyze more data points, including customer behavior, social media activity, and alternative data sources, leading to more accurate credit risk assessments. A report by Accenture highlights that AI can help lenders increase cross-selling rates by up to 20% and that AI-powered credit scoring models can be more accurate at predicting defaults than traditional credit scoring models. This benefits financial institutions by mitigating loan defaults and promotes financial inclusion by expanding access to credit for a wider population.
The financial markets are complex and dynamic, requiring split-second decision making. Local AI models can analyze vast amounts of market data in real time, identifying trends and opportunities for high-frequency trading. The global algorithmic trading market is projected to reach $3.56 billion by 2030. By leveraging AI for algorithmic trading, financial institutions can execute trades with incredible speed and precision, potentially achieving superior returns and minimizing risks. However, it’s crucial to remember that regulatory bodies actively monitor algorithmic trading practices to ensure fairness and stability in the financial markets.
The future of financial services is undoubtedly local. AI models localization rapidly transforms companies’ operations, offering enhanced security, improved performance, and reduced costs. As data privacy regulations evolve and the financial sector grapples with the ever-increasing threat of cyberattacks, the on-premise nature of local models will become even more critical. Furthermore, the growing adoption of open-source AI frameworks and the emergence of specialized AI hardware like TPUs are making local AI development more accessible and cost-effective for financial institutions of all sizes.
This AI democratization technology will empower a wider range of institutions to leverage its power, fostering a more innovative and competitive financial landscape.
Looking ahead, we can expect to see even more sophisticated local AI models emerge, capable of handling even more complex financial tasks and driving further advancements in wealth management, personalized financial advice, and risk management. The future of finance is local, and those institutions that embrace this transformative technology will be best positioned to thrive in the years to come.
The competitive landscape is rapidly evolving, and staying ahead of the curve requires leveraging cutting-edge technology. By partnering with a leading tech provider like rinf.tech, you gain access to a team of AI experts who can help you develop and optimize customized local AI models tailored to your unique needs.
Don’t settle for generic solutions – unlock the full potential of your data with local AI.
Exploring the immense potential of data-driven innovation within the financial services industry.
A comprehensive overview of how fintech innovations drive significant changes in the retail industry.
Examining AI’s diverse effects on finance, delving into its advantages and obstacles, aiming to understand its transformative influence and future impact on the sector.
Copyright © 2023 rinf.tech. All Rights Reserved.
Terms & Conditions. Cookie Policy. Privacy Policy.
Politica Avertizari de Integritate (RO)