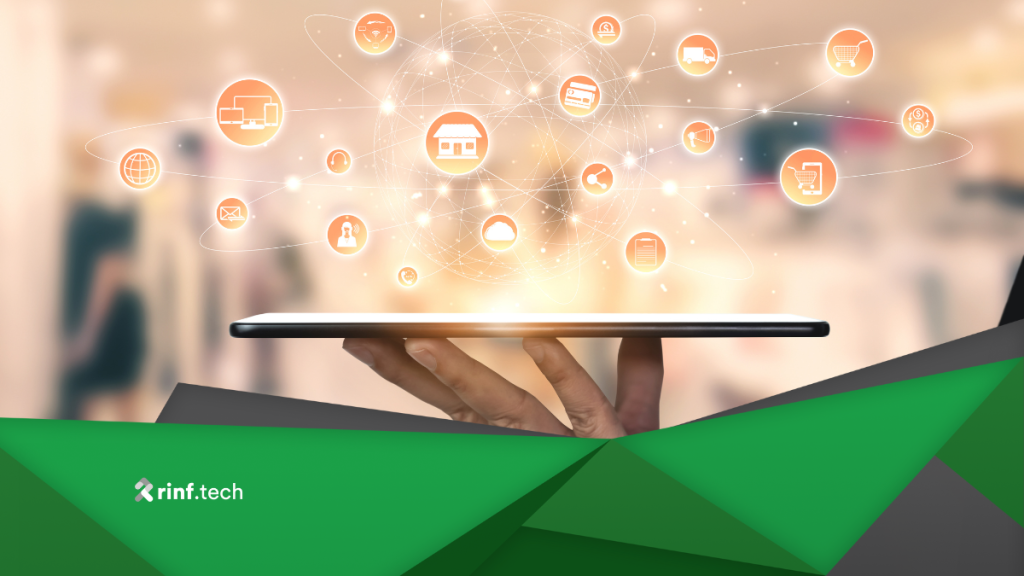
All You Need to Know About Retail Tech in 2024
In this article, we explore a current retail technology landscape and trends shaping the industry, retail tech use cases, as well as what the future holds for retail tech.
The scale of this data challenge is profound. International Data Corporation (IDC) estimates that by 2025, global data will expand to 175 zettabytes, with a substantial portion originating from supply chain and logistics systems. More critically, 90% of this data exists in unstructured formats, rendering traditional analysis methods ineffective. A Deloitte survey reveals that 60% of Chief Procurement Officers (CPOs) identify poor master data quality, lack of standardization, and weak governance as the biggest challenges in their supply chain, leading to what we call “data black holes”—large reservoirs of untapped intelligence locked within organizational silos.
Artificial Intelligence (AI) is proving to be a transformative solution to these ongoing challenges, offering unparalleled capabilities to uncover and harness the potential of these data black holes. The adoption of AI in supply chain management is advancing at a remarkable pace. A McKinsey study demonstrates that AI implementation can reduce forecasting errors by 20-50%—a game-changing capability in an environment where precision can mean the difference between operational success and significant financial risk.
This article explores how AI technologies can revolutionize supply chain data management. We will examine the challenges of data black holes, demonstrate AI’s remarkable capabilities in transforming raw data into strategic intelligence, and provide a practical roadmap for organizations seeking to harness the full potential of their data ecosystems.
The modern supply chain ecosystem represents an increasingly complex network of interconnected approaches, technologies, and global processes. Within this intricate landscape, data black holes have emerged as a critical operational challenge that fundamentally undermines organizational efficiency, strategic decision-making, and competitive agility. These data black holes are not just technical inconveniences but sophisticated systemic barriers that can significantly impair an organization’s ability to respond to market dynamics and optimize operational performance.
Multiple sources of data fragmentation contribute to the formation of these black holes. Legacy technological systems, often developed in isolation and maintained through incremental updates, create fundamental incompatibilities that prevent seamless data integration. A comprehensive study by Deloitte reveals that many enterprises maintain technology infrastructures initially designed decades ago, with some critical systems dating back to the 1990s. These outdated technologies operate on disparate data formats, communication protocols, and storage mechanisms, making holistic data analysis extraordinarily challenging.
Organizational silos represent another deep source of data black holes. Different departments—procurement, logistics, inventory management, and sales—frequently maintain separate data repositories with limited cross-functional visibility. This fragmentation creates significant blind spots in understanding end-to-end supply chain dynamics.
The consequences of these data black holes extend far beyond operational inefficiencies. They directly impact critical business metrics such as demand forecasting accuracy, inventory optimization, and risk management. According to a 2023 McKinsey global supply chain report, companies struggling with data integration challenges face substantial economic implications: approximately 18-25% higher operational costs, increased inventory holding expenses, and reduced ability to predict and mitigate potential supply chain disruptions. External data complexity further aggravates these challenges. The exponential growth of global supply networks, characterized by multiple international suppliers, complex regulatory environments, and rapidly changing geopolitical landscapes, introduces additional layers of data variability.
AI represents a transformative technological paradigm that offers unprecedented capabilities for infiltrating and decoding complex data landscapes in supply chain management. Unlike traditional analytical approaches, AI technologies provide dynamic, adaptive intelligence that can navigate the intricate nuances of massive, fragmented datasets with remarkable precision and depth.
Machine Learning (ML) is at the forefront of AI-driven supply chain insights, offering sophisticated predictive capabilities that fundamentally reshape demand forecasting methodologies. Advanced ML algorithms can analyze historical transaction data and incorporate external variables such as economic indicators, seasonal trends, and even social media sentiment to generate predictive models accurately. A landmark study by McKinsey demonstrates that ML-powered demand forecasting can reduce errors by up to 50%, potentially translating to millions in saved inventory and logistics costs for large enterprises.
Deep Learning technologies introduce an additional layer of analytical sophistication, specializing in anomaly detection and complex pattern recognition. These neural network-based systems can process vast volumes of multidimensional data, identifying subtle and often imperceptible patterns that traditional statistical methods would miss. Deep learning enables organizations to detect potential disruptions, predict equipment failures, and optimize routing strategies with unprecedented granularity in supply chain contexts.
Natural Language Processing (NLP) is a powerful tool for extracting strategic insights from unstructured text data. NLP technologies can transform previously inaccessible qualitative information into quantifiable strategic intelligence by analyzing customer feedback, social media conversations, supplier communications, and regulatory documents. Companies implementing advanced NLP in their supply chain intelligence systems can improve market responsiveness significantly and enhance supplier relationship management through more nuanced sentiment analysis.
Computer Vision is another groundbreaking AI technology revolutionizing supply chain operations. By leveraging advanced image recognition and ML algorithms, computer vision systems can automate complex visual inspection processes, monitor inventory levels in real-time, and accurately detect potential quality issues.
Generative AI in Supply Chains
The convergence of artificial intelligence and supply chain management represents a pivotal moment in organizational strategy, where data-driven insights translate into tangible operational advantages. AI emerges as a transformative force, enabling organizations to move beyond historical data constraints and embrace a proactive, intelligent approach to supply chain optimization.
Inventory management is a critical field where AI demonstrates its most profound impact. AI-powered systems can rapidly generate inventory forecasts by synthesizing complex datasets, including seasonal variations, market trends, geopolitical factors, and real-time consumption patterns. The intelligence goes beyond simple forecasting. These advanced systems can dynamically adjust inventory levels in real time, anticipate potential supply disruptions, and recommend optimal stock quantities across multiple geographical locations.
Logistics represents another field where AI introduces revolutionary capabilities. Traditional transportation management has been historically constrained by limited visibility and reactive decision-making. AI disrupts this paradigm by processing massive, multidimensional datasets that include GPS tracking, weather patterns, traffic conditions, carrier performance histories, and fuel price fluctuations. These sophisticated algorithms can generate routing strategies that optimize efficiency, reduce costs, and minimize environmental impact with unprecedented granularity.
Supply chain risk management has become a critical organizational capability in an increasingly volatile global environment. Artificial intelligence introduces a paradigm shift from reactive risk mitigation to proactive risk intelligence. By analyzing complex datasets from geopolitical sources, economic indicators, supplier performance histories, and global news streams, AI can identify potential disruptions before they materialize, enabling organizations to develop more robust, adaptive strategies. These systems generate comprehensive risk scenario models beyond traditional linear forecasting, providing nuanced, multidimensional insights that allow more strategic decision-making.
Through AI-powered intelligence, supplier management evolves from transactional interactions to strategic partnerships. Organizations can make more informed, holistic decisions about supplier selection, development, and collaboration by aggregating and analyzing comprehensive performance data across multiple dimensions. These advanced systems generate sophisticated supplier scorecards that consider not just traditional metrics like cost and delivery performance but also factors such as innovation potential, sustainability practices, and long-term strategic alignment.
Amazon, one of the world’s largest online retailers, provides a notable example of how to apply Artificial Intelligence at scale to transform supply chain management. With the help of AI, Amazon’s supply chain is speeding up by 75%. Leveraging sophisticated ML algorithms, the company continually refines its demand forecasts by analyzing massive volumes of data, including historical sales patterns, seasonal trends, local shopping behaviors, and external market indicators. These AI-driven insights enable Amazon to optimize inventory placement across its vast network of global fulfillment centers.
Walmart, another retail giant, demonstrates how AI-driven predictive analytics can streamline complex, large-scale logistics operations and inventory management strategies. With the challenge of maintaining optimal stock levels across thousands of stores, Walmart has integrated machine learning models to forecast item-level demand, accounting for local events, weather patterns, and economic indicators. In addition, Walmart leverages NLP-driven sentiment analysis to gather insights from customer feedback, enabling it to identify and address operational pain points more effectively.
DHL, a global leader in logistics, uses AI to optimize routing through dynamic route planning that adjusts to real-time traffic conditions, weather, and delivery schedules. Their AI systems analyze historical shipping data to predict potential delays, allowing for proactive rerouting and re-planning. This not only reduces delivery times but also significantly cuts down fuel consumption and operational costs, enhancing both efficiency and sustainability in their supply chain operations.
The successful implementation of AI in supply chain management begins with establishing well-defined, measurable objectives that align with overarching business strategies. Rather than embarking on AI initiatives haphazardly or merely following industry trends, companies should start by identifying the specific outcomes they want to achieve. Setting clear objectives guides the selection of appropriate AI tools and data sources and provides a benchmark for measuring success.
Determining where your most valuable supply chain data resides is critical in making AI work effectively. This may include data stored in enterprise resource planning (ERP) systems, warehouse management systems (WMS), transportation management systems (TMS), IoT sensor feeds, and even third-party vendor platforms. It’s essential to take a comprehensive inventory of these sources and understand their structure, accessibility, and relevance to your objectives. By pinpointing the right data, companies can ensure that their AI models are built on accurate, timely, and context-rich information.
No matter how advanced the AI model is, it can only perform as well as the quality of the data fed into it. Before deploying AI, organizations must focus on cleaning, standardizing, and validating their data to eliminate errors, redundancies, and inconsistencies. Equally important is safeguarding this critical asset. As supply chains increasingly rely on digital technologies and sensitive information, implementing rigorous cybersecurity measures, compliance protocols, and data governance frameworks becomes a non-negotiable requirement. Ensuring data integrity not only protects against breaches and regulatory penalties but also instills confidence in AI-generated insights. This way, companies can trust that their AI-driven recommendations are reliable and secure, facilitating better decision-making and long-term sustainability.
Effective AI solutions often require a blend of internal domain knowledge and specialized technical expertise. While some companies may hire in-house data scientists, machine learning engineers, and AI strategists, others may find collaborating with external partners or technology providers beneficial. This flexible “augmented” team structure can offer access to cutting-edge AI platforms, training services, and best practices without overburdening internal resources. Moreover, building a team that combines IT professionals, supply chain experts, and AI specialists ensures that technical innovations are always grounded in real-world business context. Over time, this synergy fosters a culture of continuous improvement, allowing the company to refine its AI models, address emerging challenges, and capture new growth opportunities.
Selecting the right technologies can be daunting with a constantly evolving landscape of AI tools, frameworks, and platforms. The key is to ensure that the chosen solutions address your specific business goals, integrate seamlessly with existing IT infrastructures, and fit your organization’s technical maturity level. It’s also crucial to consider scalability, vendor support, and interoperability with future technologies—such as GenAI—that may arise as the company’s AI journey unfolds. Enterprises can avoid costly missteps and maximize returns by carefully evaluating and selecting appropriate AI solutions.
Adopting AI in the supply chain isn’t just about integrating new technologies. It’s about transforming the organizational mindset. Creating a data-driven culture involves encouraging employees to trust AI-generated insights, embrace analytical thinking, and make informed decisions based on evidence rather than intuition alone. Leadership must lead by example, championing data literacy and continuous learning. Providing training, workshops, and professional development opportunities helps team members understand how to interpret and act on AI insights. Over time, a data-driven culture cultivates resilience, adaptability, and innovation—qualities that enable organizations to overcome challenges in an increasingly complex supply chain landscape and remain agile as market conditions evolve.
In an era where supply chains span continents, markets shift with unprecedented speed, and customer expectations continue to rise, the ability to transform obscure “data black holes” into actionable insights has become a decisive competitive advantage. We can anticipate a future where autonomous vehicles streamline deliveries, predictive maintenance virtually eliminates equipment downtime, and intelligent platforms optimize procurement cycles in near real-time—all supported by the next generation of AI-driven analytics. This is not merely a vision of what might be possible; companies are already piloting these solutions, paving the way for more robust, transparent, and purpose-driven supply chains.
By embracing AI, organizations can proactively shape their future, strengthening their operational footing in volatile markets and ensuring they are well-prepared to meet tomorrow’s challenges.
Let’s talk.
In this article, we explore a current retail technology landscape and trends shaping the industry, retail tech use cases, as well as what the future holds for retail tech.
Exploring how automation technology can solve the biggest challenges in supply chain, delivery and logistics.
Exploring the complex world of healthcare supply chain management and highlighting the importance of automation.