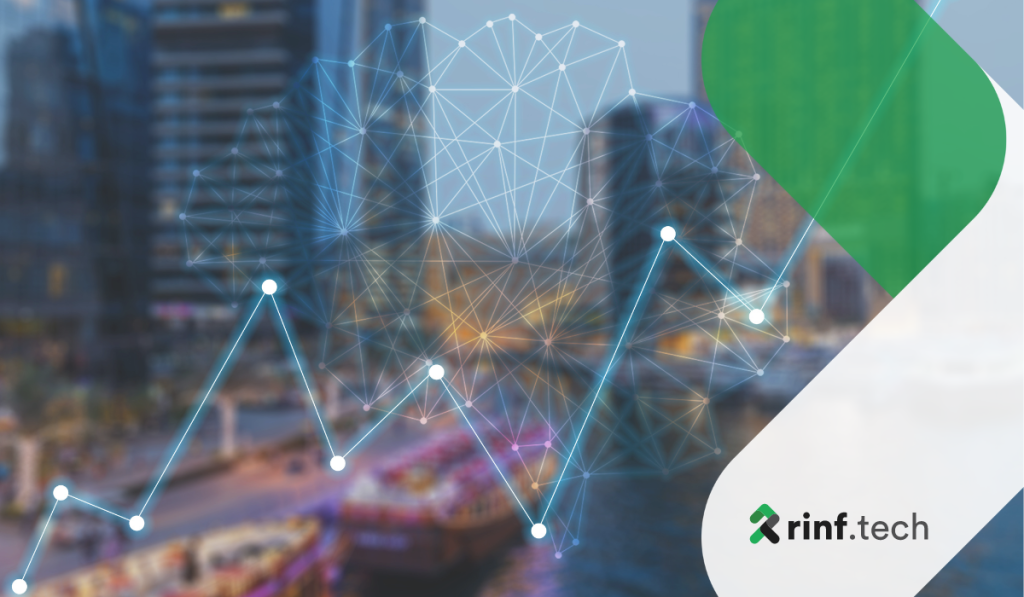
How AI and IoT Integration Creates Smarter Ecosystems
Exploring how AI IoT development impacts smart homes, smart manufacturing, and smart cities.
This synergy enhances efficiency, reduces costs, and offers innovative solutions across multiple sectors. The global AIoT market, valued at $126.1 billion in 2023, is projected to reach $1,319.4 billion by 2032, driven by the adoption of IoT devices, which number is expected to exceed 30.9 billion units by 2025.
AIoT’s impact is profound. In healthcare, it enables advanced medical devices and remote monitoring, improving patient care and efficiency. In manufacturing, AIoT facilitates predictive maintenance and optimized production, enhancing productivity and reducing downtime. In smart cities, AIoT applications improve urban infrastructure, traffic management, and public services, leading to more sustainable urban living.
Developments in IoT and AI technology, such as edge computing and advancements in machine learning and deep learning, have accelerated AIoT growth. However, challenges exist. Data privacy and security concerns are paramount due to the increased volume of sensitive information. Interoperability issues between different IoT systems and ethical considerations related to bias in AI algorithms also pose significant limitations. These challenges require a comprehensive understanding of the AIoT landscape.
This article delves into the complexities of AIoT, exploring its core principles, current state, challenges, and future trends. As AIoT continues to advance, it holds immense promise for a smarter, more connected world.
AIoT integrates AI technologies with IoT infrastructure, creating a system where connected devices can process and analyze data to make intelligent decisions autonomously. IoT involves a vast network of devices communicating and sharing data over the internet. These devices range from simple sensors to complex machinery, all generating significant amounts of data. AI, mainly through machine learning and deep learning, processes this data to extract insights and facilitate real-time decision-making.
At the heart of IoT are the numerous connected devices that, embedded with sensors and actuators, collect and transmit data across networks. These devices are pivotal in industries like manufacturing, healthcare, and smart cities, where data collection is crucial for operational efficiency and innovation.
AI technologies, including machine learning and deep learning, are essential for analyzing the massive datasets generated by IoT devices. AI algorithms process this data to identify patterns, predict outcomes, and make decisions without human intervention. The advancements in AI have been significant, with AI capabilities becoming more sophisticated and capable of handling complex tasks.
In AIoT devices, artificial intelligence is integrated into infrastructure components, such as programs and chipsets, which are interconnected through IoT networks. Application programming interfaces (APIs) are then employed to ensure seamless operation and communication among all hardware, software, and platform components, requiring no effort from the end user.
Once operational, IoT devices create and gather data, which AI then analyzes to provide insights and enhance efficiency and productivity. These insights are derived through AI systems using processes such as data learning. AIoT systems are primarily configured in either cloud-based or edge-based setups.
Cloud-based AIoT involves managing and processing data from IoT devices using cloud computing platforms. Connecting IoT devices to the cloud is essential because it is where data is stored, processed, and accessed by various applications and services. The architecture of cloud-based AIoT is composed of four layers: the device layer, the connectivity layer, the cloud layer and the user communication layer.
AIoT data can also be processed at the edge, meaning the data from IoT devices is processed as close to these devices as possible to minimize the bandwidth required to move data and avoid potential delays in data analysis. The architecture of edge-based AIoT consists of three layers: the collection terminal layer, the connectivity layer and the edge layer.
Understanding the current state of AIoT provides insight into its potential and the limitations that must be addressed to fully leverage its capabilities.
The integration of AIoT in wearable technology is redefining personal health, safety, and lifestyle. Wearables such as smartwatches, fitness trackers, and smart clothing are equipped with sensors that collect data on physical activity, heart rate, sleep patterns, and more. AI algorithms analyze this data in real time, providing users with actionable insights and personalized health recommendations. This technology not only enhances personal health management but also supports remote patient monitoring, allowing healthcare providers to track patients’ conditions outside the clinical setting.
AIoT plays a key role in developing smart cities, where technology is used to enhance the efficiency and livability of urban environments. Examples of AIoT applications in smart cities include:
These innovations lead to reduced energy consumption, lower emissions, and improved public services.
The healthcare industry is leveraging AIoT to enhance patient care and operational efficiency. AI-powered medical devices and remote patient monitoring systems allow for continuous health monitoring and early detection of medical conditions. For instance, wearable devices can track vital signs and alert healthcare providers to potential health issues in real-time. AI algorithms analyze electronic health records and medical imaging data to support accurate diagnoses and personalized treatment plans.
AIoT is transforming the manufacturing sector through predictive maintenance and optimized production processes. By collecting and analyzing data from machinery sensors, AI algorithms can predict equipment failures before they occur, allowing for proactive maintenance and reducing downtime. Additionally, AIoT systems optimize production lines by analyzing real-time data, improving efficiency and quality control.
AIoT is driving significant advancements in agriculture through precision farming techniques and crop yield optimization. AIoT systems use data from soil sensors, weather stations, and drones to monitor crop health, soil conditions, and weather patterns. This data-driven approach enables farmers to make informed decisions, optimize resource usage, and increase crop yields.
In the financial sector, AIoT enhances fraud detection, supply chain finance, and digital payments. AI algorithms analyze real-time transaction data to identify and prevent fraudulent activities. At the same time, IoT devices provide real-time visibility into the movement of goods and finances. Digital payment systems leverage AIoT for secure and efficient transactions.
The retail industry is experiencing a transformation with AIoT applications in inventory management, smart stores, and supply chain optimization. AI algorithms analyze data from IoT devices to manage inventory levels, predict demand, and optimize supply chains. Smart stores use AIoT to enhance the shopping experience through personalized recommendations and automated checkouts.
AIoT is revolutionizing transportation with applications in software-defined vehicles, smart traffic management, logistics optimization, and fleet management. AI algorithms process data from sensors and cameras to enable autonomous driving, optimize routes, and manage fleets more efficiently. Smart traffic management systems use AIoT to reduce congestion and enhance safety.
In the energy sector, AIoT supports the development of smart grids, renewable energy integration, energy efficiency, and energy distribution. AI algorithms analyze data from IoT devices to optimize energy production, distribution, and consumption. Smart grids use AIoT to balance supply and demand, integrate renewable energy sources, and improve reliability.
Edge computing represents a significant advancement in the AIoT landscape, addressing the limitations of traditional cloud computing by processing data closer to where it is generated. This technology reduces latency, conserves bandwidth, and enhances the ability of AIoT systems to provide real-time insights and actions. By processing data at the edge of the network, near the IoT devices, edge computing enables faster decision-making, which is critical for applications requiring immediate responses, such as autonomous vehicles, industrial automation, and healthcare monitoring.
Edge computing also enhances data security and privacy by minimizing the need to transfer sensitive data to centralized cloud servers, reducing the risk of data breaches and cyber-attacks. This decentralized approach is particularly beneficial for industries with strict data privacy regulations, such as healthcare and finance. The integration of AI with edge computing further amplifies its capabilities, allowing for more sophisticated analytics and autonomous decision-making at the edge, thus making AIoT systems more efficient and reliable.
The continuous advancements in AI algorithms, particularly in machine learning and deep learning, are driving the evolution of AIoT. These advancements have significantly enhanced the ability of AI systems to analyze complex datasets, recognize patterns, and make intelligent decisions. Machine learning algorithms, such as reinforcement learning and supervised learning, have improved the accuracy and efficiency of predictive analytics, anomaly detection, and pattern recognition in AIoT applications.
Deep learning, a subset of machine learning involving many layers of neural networks, has made substantial strides in processing unstructured data such as images, audio, and text. This capability is crucial for applications like computer vision in autonomous vehicles, natural language processing in smart assistants, and image analysis in medical diagnostics.
Moreover, advancements in AI algorithms have enabled more efficient model training, reduced computational requirements, and improved the interpretability of AI systems. These improvements are essential for the scalability and deployment of AIoT solutions, allowing them to operate more effectively in real-world environments.
The evolution of IoT device capabilities is another critical factor driving the advancement of AIoT. Improvements in sensor technology, connectivity, and battery life have enhanced the performance and reliability of IoT devices, enabling more complex and varied applications. Advanced sensors now offer higher accuracy, greater sensitivity, and better durability, making them suitable for a wide range of environments and conditions. For instance, new-generation environmental sensors can detect minute changes in air quality, while biomedical sensors can monitor vital signs with high precision.
Enhanced connectivity options, including the adoption of 5G technology, have significantly increased the data transfer rates and reduced latency for IoT devices. This improvement is crucial for applications that require real-time data exchange, such as autonomous driving, industrial automation, and telemedicine. The rollout of 5G is expected to further boost the capabilities of AIoT by providing faster and more reliable communication between devices, thus supporting more complex and data-intensive applications.
Battery life advancements have also played a pivotal role in the development of IoT devices. New battery technologies and energy-efficient designs have extended the operational life of IoT devices, reducing the need for frequent recharging or replacement. This is particularly important for remote or hard-to-access devices, such as environmental sensors in agriculture or monitoring devices in smart cities
One of the most significant challenges facing AIoT adoption is ensuring data privacy and security. The vast amounts of data generated by IoT devices include sensitive information such as personal health records, financial transactions, and operational data from critical infrastructure. This data is often transmitted over networks and processed by AI systems, making it vulnerable to cyberattacks, breaches, and unauthorized access. According to a report by the Internet Society, the increasing number of connected devices expands the attack surface, making it more challenging to secure these devices and the data they collect and transmit. A new report from Cisco AppDynamics revealed that 89% of global IT professionals believe their organization has experienced an expansion in its attack surface over the last two years.
Data breaches can have severe consequences, including financial losses, reputational damage, and legal liabilities. For instance, the healthcare sector, which relies heavily on AIoT for patient monitoring and medical data analysis, is particularly vulnerable to cyber threats. The average healthcare data breach has reached $10.93 million, an 8% jump from a year ago when the average cost reached $10 million for the first time. To mitigate these risks, organizations must implement comprehensive security strategies, including encryption, secure communication protocols, regular security audits, and adherence to data privacy regulations such as the General Data Protection Regulation (GDPR) and the California Consumer Privacy Act (CCPA).
Interoperability is another significant challenge in the AIoT ecosystem. IoT devices from various manufacturers often use different communication protocols, data formats, and standards, leading to compatibility issues. This lack of standardization can hinder the seamless integration of devices and systems, reducing the efficiency and effectiveness of AIoT solutions.
To address interoperability challenges, industry stakeholders must collaborate to develop and adopt universal standards and protocols. Initiatives like the Open Connectivity Foundation (OCF), which aims to create a set of standard communication protocols, are examples of how industry collaboration can lead to significant improvements in interoperability. Additionally, adopting open-source platforms and interoperability testing can help identify and resolve compatibility issues early in the development process.
Ethical considerations and bias in AI algorithms present another set of challenges for AIoT implementation. AI systems are trained on large datasets, and if these datasets contain biased or unrepresentative data, the resulting algorithms may perpetuate or even amplify existing biases. This can lead to unfair or discriminatory outcomes, particularly in sensitive applications such as hiring, lending, and law enforcement. A study by the AI Now Institute highlighted that biased AI systems could disproportionately affect marginalized groups, leading to ethical and social justice concerns.
Addressing bias in AI requires a multi-faceted approach:
Scalability and infrastructure limitations are also critical challenges for the widespread adoption of AIoT. Deploying AIoT solutions on a large scale requires robust infrastructure, including high-speed connectivity, data storage, and processing capabilities. Many regions, particularly in developing countries, lack the necessary infrastructure to support extensive AIoT deployments. This digital divide can inhibit the adoption of AIoT technologies and deepen existing inequalities.
Investments in infrastructure, such as expanding broadband access, upgrading data centers, and developing edge computing capabilities, are essential to overcome these limitations. Public-private partnerships can play a crucial role in funding and implementing these infrastructure projects. Additionally, adopting scalable cloud-based solutions can help organizations manage and process large volumes of data without extensive on-premises infrastructure.
AIoT is steering in a new era of technological advancements and opportunities across various sectors. As AIoT continues to evolve, it plays a crucial role in driving digital transformation, promoting sustainability, and enabling innovative applications.
AIoT is a vital enabler of Industry 4.0, the fourth industrial revolution characterized by the integration of digital technologies into manufacturing and industrial processes. AIoT facilitates the seamless connection of machinery, systems, and humans, allowing real-time data exchange and intelligent decision-making. This integration leads to smarter factories, where predictive maintenance reduces downtime, and automation enhances productivity and efficiency.
AIoT is poised to play a significant role in promoting sustainability and environmental monitoring. Through environmental monitoring systems, AIoT can track air and water quality, detect pollution sources, and monitor natural habitats. These systems use data from IoT sensors combined with AI analysis to provide real-time insights and facilitate proactive measures to protect the environment. For example, AIoT can optimize resource usage in agriculture through precision farming techniques, reducing water consumption and minimizing the use of fertilizers and pesticides.
Green initiatives, such as smart grids and renewable energy integration, also benefit from AIoT. Smart grids use AI algorithms to balance energy supply and demand, integrate renewable energy sources efficiently, and reduce energy losses. Additionally, AIoT enables the development of smart buildings that optimize energy consumption, enhance citizen comfort, and reduce carbon footprints.
Biometric authentication and identity management are becoming increasingly important in the AIoT ecosystem. AIoT systems leverage biometric data—such as fingerprints, facial recognition, and voice patterns—to provide secure and convenient authentication methods. These technologies enhance security by reducing the reliance on passwords and other traditional forms of authentication susceptible to breaches.
Integrating AR and VR with AIoT opens new possibilities for immersive and interactive experiences. AIoT can enhance AR/VR applications by providing real-time data and contextual information, creating more realistic and engaging environments. In manufacturing, AR can be used for remote maintenance and training, where workers use AR glasses to receive real-time instructions and visual aids overlaid on physical equipment.
AIoT offers significant disaster management and emergency response potential by providing real-time data and predictive analytics. AIoT systems can monitor environmental conditions, detect natural disasters such as earthquakes and floods, and provide early warnings to mitigate their impact. For example, AIoT-enabled sensors can detect seismic activities and alert authorities and communities to take preventive measures. In emergency response, AIoT can facilitate efficient coordination by providing real-time data on affected areas, resource availability, and the location of emergency responders.
Emerging AIoT Applications
AIoT is transforming homes and buildings into smart environments that enhance comfort, security, and energy efficiency. Smart home devices, such as thermostats, lighting systems, and security cameras, use AI to learn user preferences and optimize their operation. AIoT-enabled smart buildings can monitor and control HVAC systems, lighting, and other building functions to reduce energy consumption and improve indoor air quality.
AI-powered wearables are revolutionizing personal health management by providing continuous monitoring and personalized health insights. Wearable devices, such as fitness trackers and smartwatches, collect data on physical activity, heart rate, sleep patterns, and more. AI algorithms analyze this data to provide users with actionable health recommendations and alerts for potential health issues.
AIoT is driving the development of autonomous vehicles and intelligent transportation systems that enhance safety, efficiency, and convenience. Autonomous vehicles use AI algorithms to process data from sensors, cameras, and radar systems to navigate and make real-time decisions. AIoT also enables smart traffic management systems that optimize traffic flow, reduce congestion, and improve public transportation services.
AIoT has emerged as a transformative force, combining AI’s advanced data processing capabilities with IoT’s vast network of connected devices to revolutionize various sectors. From healthcare, where predictive maintenance and remote patient monitoring enhance patient care, to manufacturing, where AIoT-driven automation and efficiency are paramount, AIoT is driving significant improvements in productivity and quality of life. Technological advancements such as edge computing and improved AI algorithms further enhance the capabilities of AIoT, enabling real-time data analysis and smarter decision-making. However, the future potential of AIoT hinges on addressing key challenges, including data privacy and security concerns, interoperability issues, and ethical considerations in AI algorithm bias.
To fully realize the benefits of AIoT, companies must navigate these challenges by partnering with expert partners who possess the necessary technical know-how. rinf.tech can provide the expertise required to develop and implement robust AIoT solutions, ensuring successful deployment and maximizing the technology’s potential. By investing in AIoT and leveraging advancements in the field, organizations can drive innovation, improve operational efficiency, and position themselves at the forefront of the digital transformation, ready to capitalize on future opportunities and create a smarter, more connected world.
Let’s talk.
Exploring how AI IoT development impacts smart homes, smart manufacturing, and smart cities.
Exploring how embedding AI into edge devices enhances decision-making processes and revolutionizes interactions within the physical world, driving efficiencies across numerous industries.
Check out 10 actionable tips for securing your IoT ecosystem.