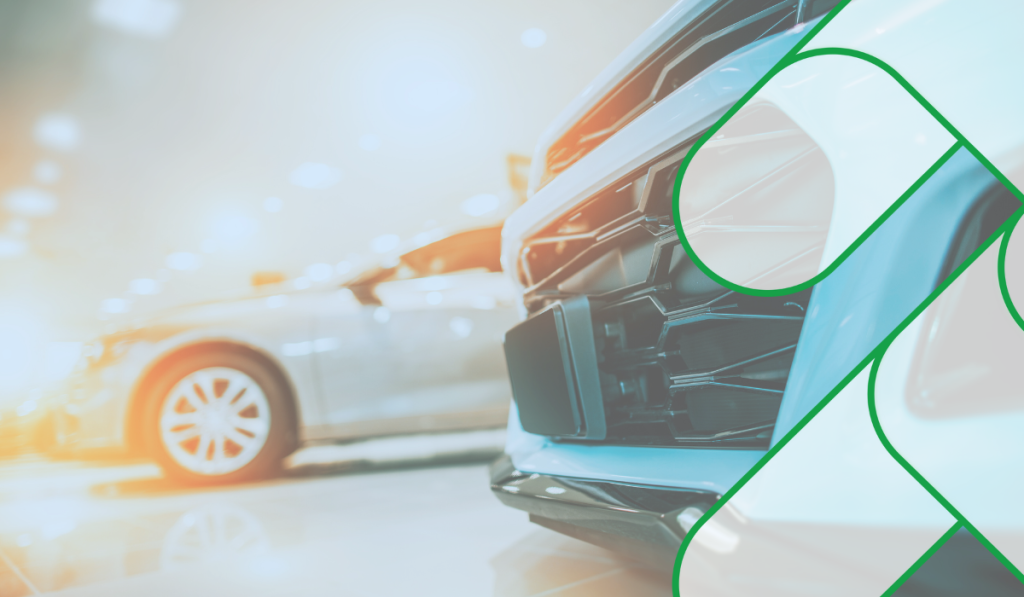
Sustainable Materials and Manufacturing in Automotive
Showcasing the environmental and economic benefits of integrating sustainable materials and robust recycling practices into the automotive value chain.
Traditional reactive maintenance approaches, which address equipment issues only after failures occur, often result in costly downtimes and inefficiencies. Studies indicate that unplanned downtime can reduce a plant’s overall productive capacity by 5% to 20%, with industrial manufacturers facing estimated losses of $50 billion annually due to these disruptions.
Predictive maintenance offers a proactive alternative by leveraging data-driven insights to anticipate and prevent equipment failures before they happen. This approach not only enhances operational efficiency but also significantly reduces unexpected interruptions. The global predictive maintenance market reflects this shift, projected to grow from $10.6 billion in 2024 to $47.8 billion in 2029.
Artificial Intelligence (AI) is at the heart of this transformation, enabling manufacturers to process vast amounts of data to make informed decisions and optimize maintenance processes. By integrating AI technologies, companies can transition from reactive to predictive maintenance models, improving equipment reliability and productivity.
This article addresses how AI technologies are transforming predictive maintenance in manufacturing.
Natural Language Processing (NLP) transforms how manufacturers extract actionable insights from unstructured data, such as maintenance logs, operator notes, and customer feedback. Traditionally, these data sources were either ignored or manually analyzed, making the process inefficient and prone to errors. NLP, however, automates this process, enabling quick and accurate analysis of large text datasets. Additionally, NLP powers voice-controlled maintenance systems that allow operators to record and retrieve data hands-free, enhancing efficiency on the factory floor.
Machine Learning (ML) is a cornerstone of predictive maintenance, providing manufacturers with the tools to analyze sensor data, identify patterns, and predict equipment failures before they occur. ML models, such as those based on supervised learning techniques like regression and classification, use historical data to predict specific outcomes, such as the likelihood of a part failing within a particular timeframe. Meanwhile, unsupervised learning techniques, such as clustering and anomaly detection, can uncover hidden patterns in data, identifying subtle changes in performance that may precede equipment failure.
Computer vision leverages image and video analysis to revolutionize equipment monitoring and defect detection. Using advanced algorithms, computer vision systems can identify anomalies, detect defects, and assess equipment conditions in real time. In safety-critical applications, computer vision can also identify hazardous conditions, such as excessive wear or overheating in machinery, providing early warnings to operators. These applications reduce reliance on human observation, improve accuracy, and accelerate response times, making computer vision a vital tool in predictive maintenance.
Deep learning, a subset of machine learning, excels in analyzing complex data and extracting intricate patterns, making it particularly suited for advanced predictive maintenance tasks. By using neural networks with multiple layers, deep learning models can process data from diverse sources—such as IoT sensors, images, and videos—to uncover relationships that simpler models might miss. Applications include predicting machinery’s remaining useful life (RUL), enabling manufacturers to schedule maintenance at optimal intervals. Deep learning also facilitates advanced anomaly detection by identifying subtle, non-linear patterns that traditional algorithms cannot detect.
AI Solutions for Predictive Maintenance In Manufacturing
Predictive failure analysis is a game-changer for manufacturers, leveraging AI algorithms to analyze historical data, real-time sensor inputs, and operating conditions to anticipate equipment failures. By processing vast datasets, AI can identify trends and patterns that signal potential breakdowns. For instance, temperature spikes, abnormal vibrations, or erratic performance data might indicate an impending failure. Early identification of such anomalies allows maintenance teams to intervene before issues escalate, minimizing unplanned downtime. This capability also optimizes maintenance scheduling, ensuring that interventions occur precisely when needed rather than on rigid schedules or after failures.
AI-powered anomaly detection is pivotal in identifying deviations from normal operating conditions, often serving as predictive maintenance’s first line of defense. These systems continuously monitor sensor readings, process parameters, and equipment behavior, flagging unusual patterns that might indicate wear, misalignment, or other issues. By catching these anomalies early, manufacturers can proactively address underlying problems, reducing repair costs and preventing potential cascading failures. Anomaly detection also ensures that even subtle issues, often overlooked by human operators, are identified and addressed promptly.
AI optimizes maintenance scheduling by integrating equipment criticality, failure probability, and resource availability. Unlike traditional maintenance schedules that are time-based or reactive, AI-driven systems dynamically adjust schedules based on real-time equipment conditions and historical performance data. This approach reduces unnecessary maintenance activities, minimizes downtime, and extends the operational life of equipment. Optimized scheduling enhances resource allocation, allowing maintenance teams to focus on the most pressing issues without wasting time or materials on low-priority tasks.
Condition-based monitoring (CBM) uses AI to provide real-time insights into the health of machinery and equipment. By analyzing sensor data continuously, AI identifies potential issues before they escalate into significant problems. This real-time feedback allows manufacturers to implement dynamic maintenance strategies tailored to the actual condition of the equipment rather than relying on fixed schedules. CBM minimizes the risk of sudden failures, optimizes maintenance interventions, and ensures maximum equipment availability, improving overall efficiency and cost savings.
Prescriptive Maintenance
Prescriptive maintenance takes predictive maintenance a step further by identifying potential issues and recommending specific actions to resolve them. AI analyzes equipment data, failure modes, and operating conditions and provides actionable insights that guide maintenance teams on the best action. This targeted approach reduces repair costs, improves maintenance efficiency, and enhances equipment reliability. Prescriptive maintenance also minimizes the guesswork involved in decision-making, allowing teams to focus on implementing solutions with the greatest impact.
AI-driven root cause analysis (RCA) is essential for identifying the underlying factors contributing to equipment failures. By analyzing historical data, AI can trace patterns and correlations to pinpoint the root causes of recurring issues. For instance, a consistent failure in a production line may be linked to a specific process parameter, material defect, or operating condition. Identifying these root causes enables manufacturers to implement long-term rather than temporary fixes, improving overall equipment reliability. RCA also reduces the frequency of recurring failures, saving costs and enhancing operational stability over time.
Energy efficiency optimization is a critical application of AI in predictive maintenance, focusing on reducing energy consumption and promoting sustainability. By analyzing equipment performance data, AI identifies inefficiencies such as excessive energy use or suboptimal operating conditions. Optimizing energy consumption reduces operational costs and aligns with sustainability goals, helping manufacturers reduce their carbon footprint and meet regulatory requirements for energy efficiency.
AI-powered predictive maintenance significantly improves equipment uptime by anticipating and preventing failures before they occur. Traditional maintenance approaches often involve either reactive repairs after breakdowns or scheduled maintenance that might not align with equipment needs, leading to unnecessary downtimes. In contrast, predictive maintenance ensures machines operate at peak performance for extended periods, reducing disruptions and increasing productivity. For instance, manufacturing plants utilizing AI for predictive maintenance have reported up to a 30% improvement in equipment uptime. This enhanced reliability allows businesses to consistently meet production targets, improving customer satisfaction and revenue streams.
AI-powered systems help reduce unnecessary maintenance activities and associated costs by targeting maintenance efforts precisely where and when needed. Traditional methods often involve routine inspections and part replacements based on predefined schedules, which can result in over-maintenance or under-maintenance. Predictive maintenance eliminates these inefficiencies by focusing resources only on equipment that genuinely requires attention. This targeted approach lowers repair costs, extends the lifespan of assets, and reduces the need for spare parts inventory.
Unplanned equipment failures can create hazardous situations, jeopardizing worker safety and leading to costly accidents. AI-powered predictive maintenance mitigates these risks by identifying potential issues before they escalate into critical failures. Predictive maintenance protects employees and reduces company liability and compliance risks by preventing accidents and ensuring safer working conditions, contributing to a safer and more secure industrial environment.
Efficient resource management is a critical benefit of AI-powered predictive maintenance. By providing real-time insights into equipment health, AI enables maintenance teams to prioritize tasks based on urgency and importance. This ensures that skilled personnel, tools, and spare parts are allocated effectively, minimizing wastage and maximizing productivity.
AI-powered predictive maintenance fosters data-driven decision-making by providing actionable insights from real-time and historical data. Maintenance teams can analyze trends, predict equipment behavior, and make informed intervention decisions. This level of precision ensures that maintenance activities align with the actual condition of machinery, reducing guesswork and enhancing operational efficiency. Moreover, data-driven insights contribute to broader operational optimization, such as identifying bottlenecks, improving production workflows, and minimizing energy consumption.
The effectiveness of AI-powered predictive maintenance depends on data availability and quality. Poor-quality data—characterized by inaccuracies, inconsistencies, or gaps—can compromise the reliability of AI models and lead to inaccurate predictions. Some legacy systems may also lack the sensors or connectivity required to generate the necessary data for advanced analytics. Even when data is available, it may be siloed across different departments or systems, making integration challenging. Manufacturers must invest in robust data collection and management processes, including sensor upgrades, data cleaning, and centralized data repositories, to ensure the reliability and accuracy of predictive maintenance solutions.
Selecting the right AI algorithms and training models effectively is critical to implementing predictive maintenance. The vast range of machine learning and deep learning algorithms can make this selection process complex. Each algorithm has specific strengths and is suited for particular use cases—e.g., supervised learning for failure prediction or unsupervised learning for anomaly detection. Furthermore, training these models requires significant domain expertise and a large volume of labeled data, which can be expensive and time-consuming.
Integrating AI-powered predictive maintenance systems with legacy infrastructure presents a significant challenge. Many manufacturing plants operate a mix of old and new equipment with varying levels of compatibility and connectivity. Bridging the gap between modern AI solutions and traditional systems often requires custom integrations, middleware, or extensive system overhauls, which can be costly and time-intensive. Additionally, organizations must ensure that new predictive maintenance tools work seamlessly alongside existing enterprise resource planning (ERP) and asset management systems.
As predictive maintenance relies heavily on connected devices and data sharing, cybersecurity, and data privacy become paramount concerns. IoT sensors and cloud-based platforms for monitoring equipment health are vulnerable to cyberattacks, resulting in data breaches, operational disruptions, or compromised safety. Manufacturers must implement robust security measures, such as encryption, access controls, and regular system audits, to safeguard their systems. Moreover, compliance with data privacy regulations, such as GDPR, is critical, especially when dealing with sensitive operational data. Organizations must balance the benefits of predictive maintenance with the need to protect their digital assets and maintain regulatory compliance.
The successful adoption of AI-driven predictive maintenance requires a skilled workforce capable of managing and maintaining these advanced systems. However, many manufacturing organizations face a skills gap, with existing staff often lacking expertise in AI, data analytics, and advanced maintenance technologies. Training employees to understand and use AI tools effectively is essential but can be a significant investment of time and resources. Additionally, fostering collaboration between traditional maintenance teams and data scientists can be challenging due to differences in expertise and operational priorities.
Cutting-edge technologies like edge AI, federated learning, and autonomous maintenance will shape the future of predictive maintenance. Edge AI allows data processing directly on devices, such as sensors and IoT-enabled machines, reducing latency and reliance on cloud infrastructure. This makes real-time decision-making possible, even in environments with limited connectivity.
On the other hand, federated learning enables AI models to learn collaboratively across decentralized devices without transferring sensitive data, addressing privacy concerns while improving predictive accuracy. Autonomous maintenance is another promising trend, where AI systems predict failures and execute basic maintenance tasks, such as lubrication or recalibration, without human intervention.
AI will continue to play a pivotal role in the evolution of smart factories, the cornerstone of Industry 4.0. Predictive AI-powered maintenance is just one aspect of a larger ecosystem where interconnected systems optimize production, resource management, and operational efficiency. With AI’s ability to integrate predictive insights with other Industry 4.0 technologies, such as digital twins and IoT, manufacturers can create fully interconnected environments that continuously learn and adapt.
While AI will undoubtedly transform predictive maintenance, human expertise will remain critical to its success. Human-AI collaboration is essential for interpreting complex insights, validating predictions, and making strategic decisions that AI systems alone cannot handle. Additionally, maintenance teams will play a vital role in training AI models by providing domain knowledge and contextual insights that improve model accuracy. The future will likely see the rise of hybrid systems where human intuition and AI precision work in tandem, ensuring that maintenance practices are not only technologically advanced but also contextually informed and strategically aligned.
As sustainability becomes a priority across industries, AI-driven predictive maintenance will be key in promoting energy efficiency and supporting circular economy initiatives. Predictive algorithms can identify inefficiencies in equipment operation, suggest optimizations to reduce energy consumption, and extend the lifecycle of machinery through timely interventions. Predictive maintenance aligns with sustainable manufacturing practices by minimizing waste, reducing the frequency of part replacements, and improving overall resource utilization. In the future, AI will be instrumental in helping companies meet their environmental, social, and governance (ESG) goals while maintaining operational excellence.
AI-powered predictive maintenance has proven to be a transformative force in manufacturing, redefining traditional maintenance practices by shifting from reactive to proactive strategies. By leveraging cutting-edge AI technologies such as machine learning, deep learning, natural language processing, and computer vision, manufacturers can unlock unprecedented levels of efficiency, reliability, and cost-effectiveness.
The benefits are clear: increased equipment uptime, reduced maintenance costs, enhanced safety, and optimized resource allocation. While challenges such as data quality, integration complexities, and workforce training remain, these hurdles are surmountable with a structured approach and a commitment to innovation.
As we move further into the era of Industry 4.0, AI-driven predictive maintenance will play an increasingly pivotal role in enabling smart factories, supporting sustainability goals, and fostering human-AI collaboration. Manufacturers who embrace these advanced technologies today will position themselves as leaders in an ever-competitive industrial landscape, ensuring long-term success and resilience.
Let’s talk.
Showcasing the environmental and economic benefits of integrating sustainable materials and robust recycling practices into the automotive value chain.
Exploring how AI technologies can be leveraged to enhance manufacturing processes, making them more sustainable.
Exploring a hypothetical scenario in which a large manufacturing company modernizes its ERP system to unlock new opportunities for scalability, agility, and enhanced operational efficiency.