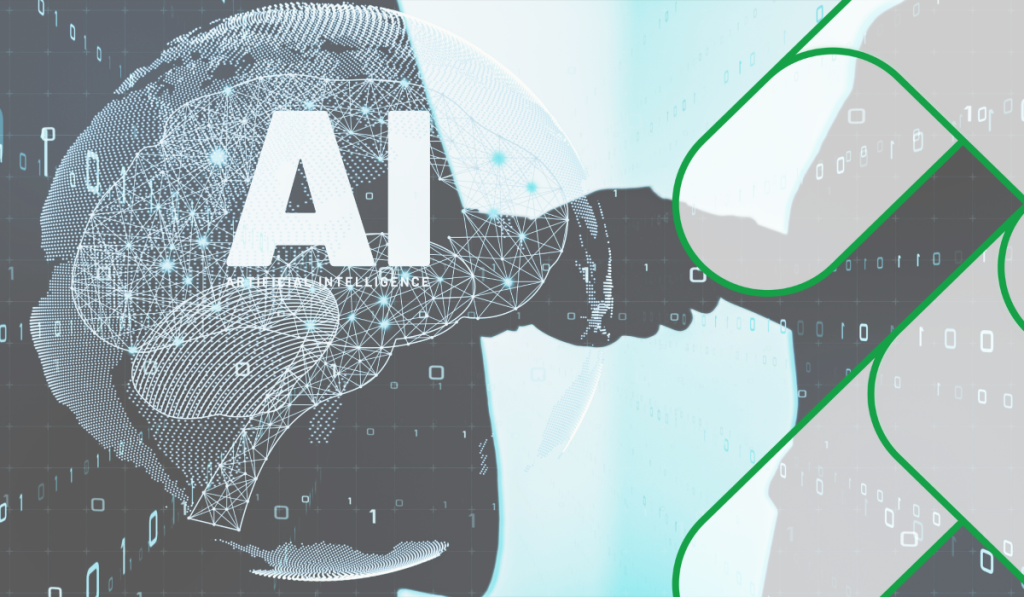
In AI, Just as in Any Tech Project, Failing Doesn’t Mean Calling It Quits
This article highlights the key reasons for AI project failures and suggests strategies for success.
Despite significant investments, over 80% of AI projects fall short of their objectives, with many never advancing beyond the pilot phase.
The high rate of AI project failures often traces back to overambitious expectations and a lack of strategic alignment. Many organizations embark on AI projects envisioning quick wins and transformative automation, only to encounter unforeseen obstacles. A notable example is IBM’s $62 million collaboration with the University of Texas M.D. Anderson Cancer Center, which failed due to inadequate planning and data management. The path to AI success requires much more than technical prowess; it demands resilience, adaptability, and a structured approach to learning from mistakes. This is where the concept of “failing fast” comes into play, encouraging organizations to embrace iterative learning, quickly identify issues, and make data-driven adjustments to their strategies.
This article highlights the key reasons for AI project failures and suggests strategies for success. It emphasizes a “fail-fast” approach for real-time learning, aligning AI initiatives with business goals, robust data strategies, cross-functional collaboration, and continuous monitoring to ensure tangible business value.
We will also examine how current factors, such as pandemic-related economic shifts, the semiconductor crisis, and the ongoing “AI bubble,” impact the long-term viability of AI investments.
The article advocates for sustainable AI applications and effective human-AI collaboration, framing AI as a strategic asset. Organizations can turn AI projects into reliable sources of sustained business value by addressing common pitfalls and embracing these best practices.
As organizations increasingly invest in AI to drive innovation and gain a competitive edge, many find themselves facing unexpected challenges that hinder success. While AI holds transformative potential, the journey from concept to implementation is often fraught with obstacles that can derail even the most promising projects. For decision makers and pioneers in AI, understanding these common pitfalls is crucial to maximizing the value of their initiatives. From setting realistic expectations and managing resources to ensuring collaboration and securing talent, this section explores the primary reasons why AI projects fail and offers insights on how to navigate these challenges effectively.
One of the most pervasive issues AI budget holders face is the need for more alignment between their expectations and AI’s practical capabilities. Organizations often launch AI projects expecting swift, transformative outcomes, only to face complex technical and operational challenges. Vague project objectives and a lack of measurable goals can exacerbate these challenges. Without clear objectives, teams may struggle to align on success metrics, making it difficult to determine whether the project is on track or valuable.
Further complicating matters is the reality of data preparation, which is both time-intensive and resource-heavy; many organizations underestimate the effort required to clean, label, and assess data quality accurately. Additionally, ethical considerations—such as potential biases in data or models—are frequently overlooked, leading to unintended consequences and compliance risks. These elements highlight the need for meticulous upfront planning, clear goal setting, and a grounded understanding of AI’s capabilities and limitations.
Financial planning for AI initiatives is often based on incomplete cost projections, especially regarding data-related expenses. The hidden costs of data acquisition, cleaning, and labeling are frequently underestimated, leading to budget overruns and, sometimes, stalled progress. Additionally, specialized talent such as data scientists, machine learning engineers, and domain experts are essential for successful AI deployment. However, the cost of hiring or training such skilled personnel is significant, and the demand for talent far exceeds supply, making skilled labor both costly and challenging to secure.
Beyond talent, AI projects require substantial ongoing investments in infrastructure for data storage, processing power, and model maintenance. These operational costs continue throughout the AI model’s lifecycle, as retraining and monitoring are essential for maintaining accuracy and relevance. When organizations neglect these resource needs, projects quickly face scalability issues, high costs, and potential failure.
The rapid pace of AI advancements means that flexibility is crucial, yet rigid project plans constrain many AI budget holders. The “fail-fast” philosophy is critical to AI, where incremental improvements and learning from early mistakes can dramatically increase a project’s chance of success. However, some organizations are slow to adapt and follow a fixed roadmap despite evolving insights or changing requirements.
Furthermore, resistance to adopting new tools, methodologies, and workflows can inhibit a team’s ability to respond dynamically to challenges. Communication barriers within AI teams often add another layer of complexity, resulting in disjointed efforts and misaligned goals. To foster agility, organizations must promote collaboration across departments, support iterative development, and prioritize adaptability in their project management approach. Without these elements, teams will experience inefficiencies, escalating costs, and project stagnation.
A successful AI project often depends on organizational support and the willingness of employees to adapt to new tools and processes. However, many organizations overlook the importance of change management and fail to secure employee buy-in, leading to resistance and limited engagement. Without clear communication, training, and incentives, employees may view AI as a threat to their roles, potentially hindering the project’s success. Addressing this challenge requires early involvement of key stakeholders and ongoing support throughout the project.
With the increasing amount of sensitive data used in AI projects, data privacy and security have become critical concerns. AI models often require access to large datasets, which may contain sensitive or personally identifiable information (PII). Organizations that neglect robust data protection protocols risk data breaches, legal liabilities, and reputational damage. Ensuring compliance with data privacy regulations like GDPR or CCPA, and implementing data encryption and access controls, are essential steps to safeguarding both data and trust.
Data is the foundation of AI, yet many organizations struggle with poor data quality and lack of infrastructure to manage it effectively. Low-quality data, which might be incomplete, outdated, or inconsistent, compromises model accuracy and can lead to unreliable outcomes. Additionally, without a robust data management infrastructure, it becomes challenging to store, organize, and retrieve data efficiently. Implementing scalable data infrastructure is vital for AI initiatives to maintain performance and accuracy over time.
AI projects often require collaboration between data scientists, domain experts, IT, and business units. However, organizations frequently encounter challenges in fostering effective communication and collaboration among these teams. Silos create inefficiencies, leading to misunderstandings, misaligned objectives, and delayed project timelines. Cross-functional collaboration, supported by clear communication channels and shared objectives, is crucial for AI initiatives to succeed.
The demand for AI expertise far outpaces supply, making it difficult for organizations to secure skilled professionals like data scientists, machine learning engineers, and AI specialists. This talent shortage limits an organization’s ability to execute and scale AI projects effectively. Training existing employees and fostering partnerships with academic and research institutions can help bridge this talent gap, but it requires significant time and investment.
AI projects consistently face higher failure rates than traditional technology projects, mainly due to distinct challenges and complexities inherent to AI. Below are the core reasons contributing to these challenges.
AI technologies rely on advanced algorithms, often involving deep learning and neural networks, which require specialized expertise and continuous refinement. Unlike more straightforward IT systems, AI projects demand a deep understanding of both the technology and the specific problem domain, making them significantly more challenging to implement successfully. This complexity introduces unforeseen technical challenges and steep learning curves, leading to delays and increased project risks.
AI’s reliance on high-quality data cannot be overstated. Success hinges on having vast quantities of relevant, well-curated data to train and validate AI models. Data quality, integration, and availability issues can derail AI projects, whereas traditional IT projects are typically less data dependent. AI systems are only as effective as the data they learn from; without rigorous data governance, organizations risk building biased or inaccurate models that fail to meet their objectives.
Many AI initiatives falter because they lack clear alignment with organizational goals. AI projects may struggle to secure ongoing support and resources without a direct connection to strategic priorities, ultimately losing momentum. Misalignment often results in AI solutions that do not address critical business needs, making it difficult to justify continued investment. Ensuring AI projects are strategically positioned helps maximize value and align stakeholder expectations with achievable outcomes.
There is often a gap between what organizations expect AI to accomplish and what is realistically achievable within their current capabilities and resources. This misalignment leads to inflated promises and can set AI projects up for failure when they inevitably fall short. Organizations may expect AI to solve complex, ambiguous challenges autonomously, while the technology may only provide incremental improvements, particularly without well-defined objectives.
Organizational cultural resistance can stall AI adoption, especially when employees fear job displacement or do not fully understand how AI will transform their roles. Without efforts to foster a positive AI culture, teams may resist adopting new systems or processes, slowing implementation and diminishing potential impact. Clear communication about AI’s role and benefits is critical to overcoming these barriers and gaining employee buy-in.
AI demands substantial infrastructure support, often underestimated in early planning. These requirements can create significant technical and financial hurdles, from computing power and data storage to integrating existing systems. Many organizations fail to anticipate the resources required for AI model training, maintenance, and deployment, leading to project bottlenecks and increased operational costs. Investing in the proper infrastructure is essential for enabling scalability and ensuring reliable, real-time AI capabilities.
If your AI program encounters any of these issues, it is essential to evaluate each project carefully. Use the recommended tool at the end of this article to customize your evaluation process and identify potential adjustments needed to keep your AI transformation program on track.
Navigating the complexities of AI implementation requires more than just technical skill—it demands agility, resilience, and a willingness to learn from missteps. Adopting a “fail fast” mindset enables organizations to minimize risks and quickly adapt to challenges, turning early setbacks into valuable insights.
In this section, we explore strategies that empower AI teams to move forward confidently, from fostering a culture of experimentation to prioritizing high-quality data and building strong cross-functional collaboration. By embracing these best practices, organizations can transform initial hurdles into stepping stones toward long-term success in AI.
The iterative approach is fundamental to AI success, allowing teams to pivot based on real-time feedback and evolving project needs. Starting with small, well-defined pilot projects is essential to this approach, enabling budget holders to test ideas without committing extensive resources prematurely. Collaborating with an experienced tech partner, like rinf.tech, can be valuable here, as they bring established R&D expertise and a structured framework for rapid iteration.
Key performance indicators (KPIs) should be established early to assess the project’s progress and determine the viability of its direction; these can include metrics such as model accuracy, processing speed, and relevance to business goals. Emphasizing rapid prototyping and testing fosters a learning culture, encouraging teams to see early setbacks as learning opportunities rather than failures. This approach enables quicker refinements and helps budget holders make informed decisions about scaling, pivoting, or abandoning a project.
High-quality data is the cornerstone of any AI initiative. Investing in data infrastructure and governance from the outset ensures that data remains clean, well-labeled, and accessible throughout the project lifecycle. Data governance frameworks help to streamline data preparation, reduce errors, and ensure compliance with regulatory standards. AI teams should also explore synthetic data generation and augmentation as cost-effective solutions for filling data gaps and enhancing model robustness.
Addressing data privacy and security concerns is equally critical; adhering to guidelines like GDPR can help protect user data and build trust in the AI system. By prioritizing data quality and accessibility, AI budget holders can reduce model errors and improve overall project outcomes, making the solution reliable and compliant with industry standards.
The complexity of AI projects demands a skilled, cross-functional team that brings technical expertise and business insight. Hiring or training highly qualified data scientists, machine learning engineers, and domain experts is crucial, as these professionals drive the technical execution of AI models. However, successful AI deployment also requires collaboration between technical teams and business stakeholders, who provide valuable context and align the AI project with organizational objectives.
Encouraging knowledge sharing and continuous learning within the team can improve adaptability and drive innovation while leveraging external partnerships, which allows organizations to tap into specialized expertise as needed. Fostering a collaborative environment helps ensure that every team member contributes to a shared vision, enabling smoother project execution and greater alignment with business goals.
Effective monitoring and evaluation ensure that AI projects remain on track and aligned with business objectives. Establishing robust monitoring systems and continuously tracking KPIs like model accuracy, user engagement, and operational efficiency allows teams to detect issues early and make timely adjustments. Regular reviews and retrospectives are valuable for assessing progress and identifying potential improvements, helping budget holders stay responsive to new insights or changes in project scope.
Furthermore, AI teams should be prepared to adapt strategies and models in response to evolving needs or market dynamics. By continuously monitoring, evaluating, and adjusting, AI budget holders can better manage resources, optimize outcomes, and navigate challenges effectively, ensuring the long-term success of their AI initiatives.
Once an AI project has “failed fast,” the next step is to regroup, analyze the lessons learned, and adjust strategies accordingly. Effective regrouping includes several essential steps to ensure future initiatives are better positioned for success.
After a project fails, a comprehensive post-mortem analysis is crucial to uncovering the underlying reasons for the failure. This process should involve collecting insights from all stakeholders—including technical teams, business leaders, and end-users—to identify both technical and strategic shortcomings. By taking a holistic approach, organizations can clearly understand where missteps occurred and use this knowledge as a foundation for improvement.
One of the most important steps in regrouping is revisiting and refining project objectives. This involves ensuring that the project’s goals align with broader business priorities and recalibrating success metrics where necessary. By redefining what success looks like, organizations can better scope future projects to meet core organizational needs and increase the chances of tangible impact.
AI projects benefit significantly from cross-functional collaboration, as involving diverse stakeholders can provide new insights and uncover potential blind spots. Bringing together experts from departments such as data science, IT, product development, and business operations can lead to a more comprehensive approach, improving project planning, resource allocation, and implementation strategies.
Lessons from a failed project should inform and improve future approaches. This might include adopting updated technologies that align more closely with the project’s requirements, refining data management practices to ensure better data quality, or enhancing team capabilities through targeted training. Organizations can reduce the risk of similar challenges recurring in future projects by adapting strategies based on insights gained.
Establishing continuous monitoring mechanisms is essential for tracking progress and identifying potential issues early in the lifecycle of future projects. By implementing tools and processes for real-time monitoring, teams can detect deviations from the project plan and make necessary adjustments before minor issues escalate. This proactive approach helps maintain momentum and contributes to a more agile and resilient project management process.
Engaging multiple stakeholders in the learning process is essential to fully benefit from a “fail-fast” approach and subsequent regrouping. Each group has a unique role, contributing to a comprehensive understanding of project challenges and driving improvements that enhance future success.
Executives are crucial in providing strategic direction and ensuring that AI projects align with the organization’s overarching business objectives. Their support is also essential in cultivating a culture that views failure as an opportunity for growth rather than a setback. By promoting an open, adaptive approach, executives can encourage teams to innovate without fear, enabling the organization to learn quickly and adjust more effectively.
As the communication bridge between teams, project managers ensure that insights and lessons learned from past projects are well-documented and accessible across the organization. They also facilitate cross-functional dialogue, connecting technical teams with business units to promote a shared understanding of challenges and successes. This role is pivotal in making sure that the insights gained from each project are captured and applied to future initiatives.
Technical teams are at the forefront of implementing improvements based on previous failures. Data scientists and engineers can leverage insights from past setbacks to refine algorithms, enhance data preprocessing methods, and improve overall model accuracy and reliability. Their focus on technical optimization addresses specific project weaknesses and elevates the organization’s AI capabilities, positioning it for more robust performance in future projects.
As individuals directly interact with AI solutions, end users offer invaluable usability, functionality, and operational impact feedback. By actively involving end users in the evaluation process, teams can gain insights into how AI tools affect day-to-day tasks and identify areas for improvement that may otherwise go unnoticed. This feedback loop is essential for ensuring that AI solutions are practical, intuitive, and aligned with real-world needs.
This tool provides a framework for decision-making, but final decisions should also consider the specific context of your organization and project.
To use it:
Realizing AI’s transformative potential requires a balanced approach: technical expertise must be paired with clear business objectives, a commitment to adaptability, and a willingness to learn from early missteps. Organizations can transform AI initiatives from high-risk ventures into strategic assets that drive sustained business value when these elements are harmonized.
AI’s rapid evolution demands proactive engagement. Groundbreaking advancements often lead to significant corrections, and the $600 billion AI challenge presents tremendous opportunities and considerable risks. Rather than stepping back, organizations should embrace a proactive stance, reprioritizing and adapting strategies to mitigate potential pitfalls while seizing new advantages. By aligning technical efforts with strategic goals, fostering a culture that views early failures as learning opportunities, and remaining agile in response to technological shifts, businesses can turn the complexities of AI into a pathway for lasting competitive advantage.
Let’s talk.
This article highlights the key reasons for AI project failures and suggests strategies for success.
Exploring how blockchain can enhance supply chain management.
Exploring how AI enables effective personalization by leveraging essential components like user data analysis, recommendation engines, dynamic interfaces, and ethical guidelines