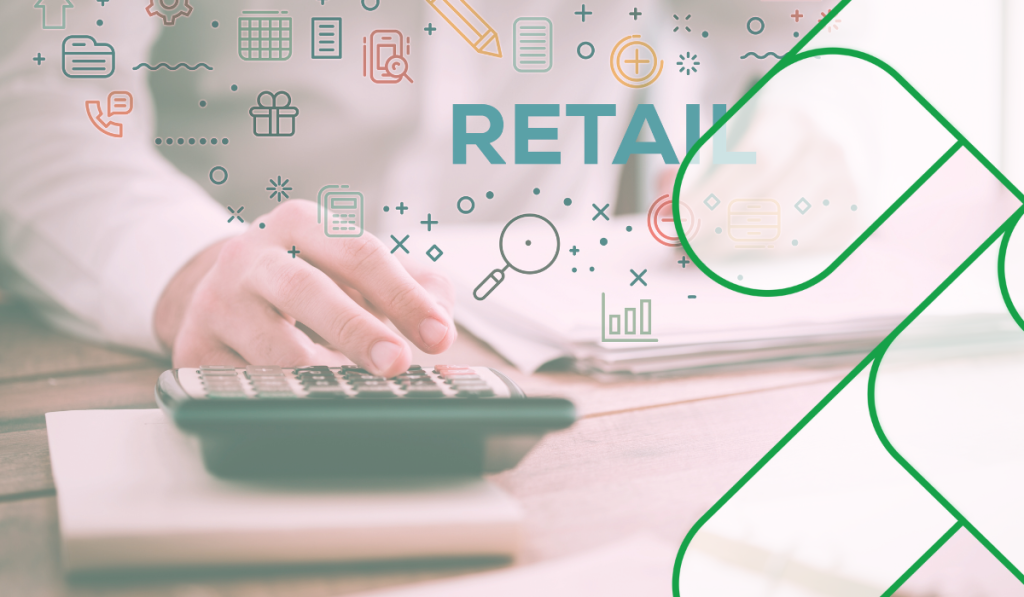
Omnichannel Retail: Technologies, Challenges, and Opportunities
This article explores omnichannel retail. It covers its advantages, the technology driving it, the implementation challenges companies face, and future trends.
In the rapidly evolving world of Retail, staying abreast of the latest trends and technologies is crucial for industry professionals. As we prepared for an important business gathering with experts from various retail specializations, we realized the need for a comprehensive yet swift method to assimilate a vast amount of industry-specific information. To meet this challenge, we turned to an advanced tool at our disposal: Large Language Models (LLM) that we had meticulously fine-tuned with an up-to-date corpus of relevant domain-knowledge material over the past months. A special embedding model was used for the information ingestion, accompanied by a custom tokenizer.
Starting from a base model, we have fine-tuned both the personalities as well as and the knowledge span for several “domain – expert” models that have received a curated corpus of information pertaining to retail strategy, technical expertise, customer satisfaction, operational intelligence, etc. All agents/domain-experts are 70B models. The main model acts as a “moderator” presenting thoughtful questions and topics to the rest of the models engaged as participants in a panel focusing on the “innovation and applications of LLM’s in the retail sector”.
This white paper delves into the multifaceted applications of LLMs in retail, examining their impact on customer engagement, operational efficiency, and decision-making processes. It serves as a guide for retail decision-makers seeking to understand and leverage the power of LLMs in their business strategies.
In the realm of artificial intelligence and machine learning, Large Language Models (LLMs) have emerged as a groundbreaking development. These sophisticated models are designed to understand, interpret, and generate human language in a way that is both meaningful and contextually relevant.
They are “large” not only in their physical size, requiring substantial computational power, but also in their capacity to handle and analyze vast datasets. These models are trained on extensive corpora of text data, enabling them to understand nuances, idioms, and the subtleties of human language.
Large Language Models function by utilizing algorithms based on neural networks, specifically a type called transformers. These transformers analyze and predict language patterns, learning from the immense datasets they are exposed to. As they process text, they pick up on linguistic structures, contextual meanings, and the varied use of language across different scenarios.
Over the years, LLMs have evolved significantly. Early models were limited in their understanding and generation of coherent text. However, with advancements in technology and machine learning techniques, modern LLMs like GPT (Generative Pre-trained Transformer) have set new standards in language understanding and generation. These models can write essays, create poetry, summarize texts, translate languages, and even generate human-like responses in conversations.
LLM integration in retail varies significantly across global markets, influenced by technological infrastructure, cultural and consumer behavior, economic factors, regulatory environment, retail sector maturity, and the local ecosystem. Understanding these variations is key for retailers looking to implement LLMs effectively in different regions.
LLMs like GPT-4 are increasingly being integrated into the retail industry. Their applications are broad and multifaceted, offering substantial benefits in various areas.
LLMs power advanced chatbots and virtual assistants that provide instant, 24/7 customer support. These systems can handle a wide range of queries, from product recommendations to troubleshooting, significantly enhancing the customer experience.
LLM-powered inventory management represents a significant advancement over traditional methods, offering a more dynamic, efficient, and responsive approach to managing retail inventory.
Aspect | Traditional Inventory Management | LLM-Powered Inventory Management |
Data Analysis | Limited to historical sales data and manual forecasting methods. | Utilizes real-time data, including market trends and customer behavior. |
Efficiency | Often time-consuming and prone to human error. | Highly efficient, automates many processes. |
Accuracy | Can be less accurate, leading to overstock or stockouts. | Highly accurate, predictive analytics reduce errors in stock levels. |
Adaptability | Slow to adapt to market changes or consumer trends. | Highly adaptable, quickly responds to market dynamics. |
Cost Effectiveness | Can be less cost-effective due to inefficiencies. | More cost-effective in the long run due to optimized stock levels and reduced waste. |
Scalability | Scaling can be challenging and resource-intensive. | Easily scalable, accommodates business growth seamlessly. |
Customer Satisfaction | May not align closely with customer needs and preferences. | Improves customer satisfaction by ensuring product availability and variety. |
Integration | Often operates in isolation from other business systems. | Integrates with other retail systems for a holistic approach. |
Innovation | Traditional methods may lag in adopting new technologies. | Encourages innovation, leveraging the latest AI advancements. |
Environmental Impact | Inefficiencies can lead to higher waste and environmental impact. | Optimized inventory reduces waste and potential environmental impact. |
Scaling Large Language Models for extensive retail operations presents several challenges.
By addressing these challenges with thoughtful and innovative solutions, LLMs can be effectively scaled to meet the demands of large retail operations, offering significant benefits in terms of efficiency, customer engagement, and business insights.
Protecting customer data in LLM-integrated retail systems is critical, and there are several key security measures that need to be implemented:
Implementing these measures can significantly enhance the security of customer data in LLM-integrated retail systems, helping to protect against breaches and ensuring compliance with data protection laws.
The role of LLMs in promoting sustainable practices and ethical AI usage underlines a commitment to social responsibility, while global market expansion and employee training and development highlight the importance of adaptability and a tech-savvy workforce. In essence, the strategic integration of LLMs in retail business strategies is a multifaceted endeavor that positions businesses for sustained growth and a competitive edge in an ever-evolving market.
Incorporating LLMs into long-term retail business strategies involves a multifaceted approach, focusing on enhancing customer experience, operational efficiency, and market adaptability, while also considering sustainability and ethical AI use. This strategic integration can position retail businesses for sustained growth and competitive advantage in a rapidly evolving market.
The success of the LLM-based chatbot as a Personalized Shopping Assistant was evident in the increased customer engagement, higher conversion rates, and improved customer satisfaction. Its ability to understand and respond to complex queries in a conversational manner was pivotal. This case study demonstrates the potential of AI and machine learning in transforming customer experiences in the e-commerce sector.
The successful implementation of an LLM-based chatbot in e-commerce requires navigating a complex array of technical challenges, from data handling to integration, contextual understanding, scalability, NLU/NLG, security, continuous learning, and user experience design. Addressing these challenges is crucial for realizing the full potential of such AI systems in enhancing customer engagement and driving sales.
Privacy and data security are paramount when implementing LLM-based chatbots in e-commerce. These considerations encompass a wide range of aspects from data collection, legal compliance, and user privacy to robust security measures and continual monitoring. Ensuring adherence to these factors is crucial for maintaining user trust and safeguarding sensitive information in e-commerce environments.
LLM-based chatbots stand out in their ability to interact conversationally with customers, offering a unique blend of personalization and real-time assistance. While other AI technologies in e-commerce like recommendation systems, inventory management tools, analytics tools, and visual search capabilities serve more specialized roles, LLM-based chatbots provide a more comprehensive and interactive customer experience. Each technology, however, plays a pivotal role in enhancing various facets of the e-commerce ecosystem.
The exploration of Large Language Models (LLMs) in the retail sector, as detailed in this white paper, underscores their transformative impact on the industry. From enhancing customer experience through personalized interactions and 24/7 chatbot support to driving data-driven decision-making with in-depth market and competitive analyses, LLMs are redefining the retail landscape. Their role in streamlining operational efficiency, aiding in inventory management, and automating routine processes further elevates their significance. Furthermore, LLMs are pivotal in fostering innovation, assisting in new product development, and enabling businesses to adapt swiftly to market changes.
The scalability of these models ensures that retail businesses are not only equipped for current demands but are also future-ready, capable of expanding and evolving with technological advancements. The commitment to sustainability and ethical AI use within these systems reflects a deeper understanding of social responsibility, vital in today’s business world. Additionally, the global market expansion facilitated by LLMs, with their ability to adapt to local languages and cultural nuances, positions retail businesses on a global stage, expanding their reach and influence.
Incorporating LLMs into retail strategies is not without its challenges, particularly in scaling these models to meet the vast and varied demands of the retail sector. Issues such as data volume, customization, real-time data integration, scalability, data privacy, and cost management require innovative and strategic solutions. However, the potential benefits, including enhanced customer satisfaction, improved operational efficiency, and increased business insights, make tackling these challenges a worthwhile endeavor.
In conclusion, the strategic integration of LLMs in retail business strategies represents a significant step towards a more efficient, customer-centric, and innovative future. By embracing these advanced AI technologies, retail businesses can position themselves for sustained growth and maintain a competitive edge in an increasingly digital and dynamic marketplace. As technology evolves, so will its applications, promising even more profound impacts on the retail industry in the years to come.
This article explores omnichannel retail. It covers its advantages, the technology driving it, the implementation challenges companies face, and future trends.
A comprehensive overview of how fintech innovations drive significant changes in the retail industry.
In this article, we explore a current retail technology landscape and trends shaping the industry, retail tech use cases, as well as what the future holds for retail tech.